Stravl.org is solely used for administrative services. Stravl's user-facing website is available at
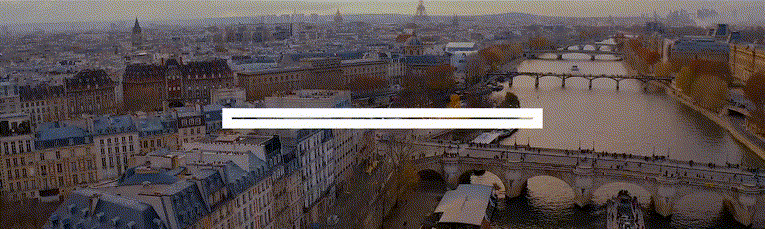
Stravl Data
Stravl is open-sourcing over 1,000,000 user travel preference data.
Gain access to Stravl's extensive travel preference data, the largest custom data set of its kind. You are free to use the data for any academic or commercial purposes. We're happy to help with questions, just reach out to [email protected] .
Stravl Data Repository
Download Stravl Data [317MB]
Optional Attribution:
Sursock, A. & Brooks, C. (2023). Stravl Travel Preference Data A. www.stravl.org/data.
Data Descriptions:
Stravl-Data is the world's largest, open-sourced data set of travel preferences. Through a user-facing website, Stravl gathered 80,301 travelers' vacation preferences. Users were asked to fill out a brief form on their ideal vacation preferences (such as expected experiences, scenery, and activity level) and logistical constraints (such as budget, traveler ages, and season). Thereafter, they were asked to "swipe" or "rate" ten destinations. Users have the option to respond with "Yes", "No", or "Maybe" in a Tinder-like rating framework. In total, over 1,000,000 user swipes were recorded. Lastly, users are shown 5 to 10 recommendations, which were selected using different ML-models. Those recommendations are recorded and users' feedback on them (through clicking a "smiley" or "frowny" face next to them) is stored. Personally-identifying information such as IP data, user names, or other metadata has been removed prior to the release of this dataset.
Stravl's extensive travel preference data is the largest custom data set of its kind. You are free to use the data for any academic or commercial purposes. We're happy to help with questions, just reach out to [email protected]. Below find a brief description of all columns in our data set.
Form Responses:
What age ranges are present in your travel group? [multiple selections possible]
What is your trip budget (per person, per night)?
2: $100-$249
What season are you planning to travel?
What are you looking to experience? Multiple selections encouraged.
1: Adventure
4: Nightlife
6: Shopping
What scenery are you seeking? Multiple selections encouraged.
3: Mountain
Activity Level
0: Chill & Relaxed
1: Balanced
Safety Conscious
0: Very Safety Conscious
2: Ready for Anything
Destination Popularity
0: Off the Beaten Path
1: Classic Spot
2: Mainstream & Trendy
Where do you want to go?
0: Anywhere
1: Specific Regions
Which specific regions? [If ‘Specific Regions’ is selected in FORM_R] [multiple selections allowed]
n: N. America
c: Caribbean
s: S. America
m: Mid. East
Swipe Responses:
Each of "yes_swipes", "no_swipes", and "maybe_swipes" includes a list of indices representing destinations a user swiped 'yes', 'no', or 'maybe' on. The indices can be transformed to its corresponding destination names through the "destination_ids" table.
Model, Recommendations, and Ratings:
Users were then recommended a set of 5 destinations (or 10 if a user wanted more). Different algorithms were used to recommend those destinations; the variables "model", "retrieval", and "dynaMatch" indicate which algorithms were used. Their implementations are not released, yet their selections are still shared for research purposes.
Each of the recommendation columns includes the names of recommended destinations in order of recommendation; if a column includes '-1' recommendations were not yet tracked at the time this user completed the form. Each rating column includes ant user ratings that were provided. If all ten columns are set to -1, ratings were not tracked at the time this user completed the form. If not, a rating of '-1' indicates a user's disapproval with the rating, and a rating of '1' indicates their approval of it. If the entry is empty, the user has not rated the corresponding destination.
For an updated version of Stravl Data, Star our GitHub repository here: https://github.com/Stravl/Stravl-Data
- About data.world
- Terms & Privacy
- © 2024 data.world, inc
Thank you for visiting nature.com. You are using a browser version with limited support for CSS. To obtain the best experience, we recommend you use a more up to date browser (or turn off compatibility mode in Internet Explorer). In the meantime, to ensure continued support, we are displaying the site without styles and JavaScript.
- View all journals
- My Account Login
- Explore content
- About the journal
- Publish with us
- Sign up for alerts
- Data Descriptor
- Open access
- Published: 23 September 2021
A database of travel-related behaviors and attitudes before, during, and after COVID-19 in the United States
- Rishabh Singh Chauhan ORCID: orcid.org/0000-0001-7188-557X 1 ,
- Matthew Wigginton Bhagat-Conway ORCID: orcid.org/0000-0002-1210-2982 2 ,
- Denise Capasso da Silva ORCID: orcid.org/0000-0003-1414-8439 3 ,
- Deborah Salon ORCID: orcid.org/0000-0002-2240-8408 2 ,
- Ali Shamshiripour 1 ,
- Ehsan Rahimi ORCID: orcid.org/0000-0002-8649-7542 1 ,
- Sara Khoeini 3 ,
- Abolfazl (Kouros) Mohammadian ORCID: orcid.org/0000-0003-3595-3664 1 ,
- Sybil Derrible ORCID: orcid.org/0000-0002-2939-6016 1 &
- Ram Pendyala 3
Scientific Data volume 8 , Article number: 245 ( 2021 ) Cite this article
8446 Accesses
12 Citations
2 Altmetric
Metrics details
- Interdisciplinary studies
- Research data
The COVID-19 pandemic has impacted billions of people around the world. To capture some of these impacts in the United States, we are conducting a nationwide longitudinal survey collecting information about activity and travel-related behaviors and attitudes before, during, and after the COVID-19 pandemic. The survey questions cover a wide range of topics including commuting, daily travel, air travel, working from home, online learning, shopping, and risk perception, along with attitudinal, socioeconomic, and demographic information. The survey is deployed over multiple waves to the same respondents to monitor how behaviors and attitudes evolve over time. Version 1.0 of the survey contains 8,723 responses that are publicly available. This article details the methodology adopted for the collection, cleaning, and processing of the data. In addition, the data are weighted to be representative of national and regional demographics. This survey dataset can aid researchers, policymakers, businesses, and government agencies in understanding both the extent of behavioral shifts and the likelihood that changes in behaviors will persist after COVID-19.
Machine-accessible metadata file describing the reported data: https://doi.org/10.6084/m9.figshare.15141945
Similar content being viewed by others
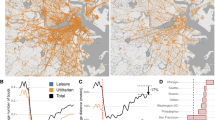
Effect of COVID-19 response policies on walking behavior in US cities
Ruth F. Hunter, Leandro Garcia, … Esteban Moro
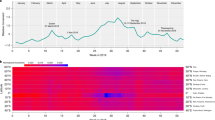
Mapping global variation in human mobility
Moritz U. G. Kraemer, Adam Sadilek, … John S. Brownstein
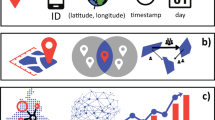
Mobility networks in Greater Mexico City
Marisol Flores-Garrido, Guillermo de Anda-Jáuregui, … Maribel Hernández-Rosales
Background & Summary
The COVID-19 pandemic has spread across the world, infecting tens of millions and killing over one million people 1 . By March 2021, the United States (U.S.) had recorded the highest number of confirmed COVID-19 cases and COVID-19 related deaths in the world 1 . Since social distancing is one of the most effective measures in containing the spread of the infection 2 , several U.S. states issued various restrictions including stay at home orders. Moreover, numerous restaurants and bars closed for dine-in services, various recreation facilities were shut down, many offices and schools switched from meeting in-person to meeting online, and travel restrictions were imposed. These measures had a profound impact on how people in the U.S. went about their daily lives.
To understand the current and future impacts of the pandemic, we conducted a nationwide online survey. The goal of the survey is to capture attitudes and shifts in travel-related choices of people across the nation both during the pandemic and once COVID-19 is no longer a threat. The data are shared publicly in order to help government agencies and businesses prepare for the future. We are conducting additional survey waves with the same respondents to monitor how people’s choices evolve over the course of the pandemic and beyond.
An early version of the survey took place from April to June 2020, when the stay at home orders were in place in most parts of the country 3 , 4 ; this portion of the data collection is referenced as Wave 1 A . A slightly-modified larger-scale survey, Wave 1B ,was deployed between late June and October 2020. Subsequent survey waves are being conducted as the situation evolves. The collected data are released as they become available and necessary procedures for cleaning, documenting, and weighting the data are completed. This procedures for data processing are detailed in this paper. The present article focuses on data from the first wave of the survey.
In the months following the beginning of the spread of COVID-19, several efforts have been made to collect data related to COVID-19. In fact, many datasets have been compiled, specifically on COVID-19 testing 5 , medical imaging of COVID-19 cases 6 , the timeline of government interventions 7 , policy announcements 8 , implementation and relaxation of public health and social measures 9 , epidemiological data 10 , mobility-related data 11 , and out-of-home activity information 12 , to name a few. Researchers also turned to social media platforms, like Twitter and Instagram, to gather COVID-19-related data 13 , 14 , 15 , 16 . Furthermore, several surveys have been conducted to measure the impacts of the pandemic 17 , 18 , 19 , some of which are now released for public use 20 , 21 .
Our survey data are different from most others in several ways. First, it is comprehensive insofar as it includes data about a wide range of topics including commuting, daily travel, air travel, working from home, online learning, shopping, attitudes, risk perception, and socioeconomic and demographic details. Second, it captures detailed information about behaviors before and during the COVID-19 pandemic, as well as the choices that people expect to make when the COVID-19 virus is no longer a threat. Third, it was collected from respondents across the U.S., covering diverse socio-economic backgrounds, professions, education levels, and ages. Fourth, the survey is a true longitudinal panel survey, collecting data in multiple waves from the same individuals at regular intervals. Finally, the data are made publicly available to promote data-driven analysis and research.
The next section describes the data collection methodology, the questions included in the survey, the survey deployment process, and the participant recruitment strategy. Next, the data records section describes the data file types and metadata. Subsequently, the technical validation section explains the procedure for the survey data cleaning and weighting. Lastly, the final section provides additional notes for data users.
Ethical compliance
Our study protocol was approved by both Arizona State University (ASU) and University of Illinois at Chicago (UIC) Institutional Review Board offices. Participants were informed that their participation is voluntary, and that their responses are shared anonymously. An online informed consent was obtained from everyone who responded to the survey.
Survey questions
The data were collected through an extensive online survey with over 120 questions. The survey questions can be broadly divided into three categories: (1) retrospective questions focusing on the period before COVID-19, (2) questions about the period during COVID-19, and (3) prospective questions on respondent expectations for a future period in which COVID-19 is no longer a threat. The questions cover a wide variety of subjects including commuting habits, discretionary travel choices, work-related questions, study-related questions, shopping, dining, and so on – all before, during, and expected after the pandemic.
The survey questions can be classified into eight categories based on question subject type, namely: demographics, work, study, shopping and dining, transportation, and general attitudes. Table 1 describes each of these categories.
Survey recruitment
From April to mid-June 2020, initial Wave 1A responses were collected from a convenience sample via mailing lists, social media outreach, and mainstream media articles. A total of 1,110 responses were collected during this phase.
From late June onward, Wave 1B, the modified version of the survey, was deployed through survey invitations sent to a random email list purchased from a data marketing company. The list contained 350,000 email addresses belonging to people in 24 metropolitan areas across the U.S., as well as the state of Ohio (see Fig. 1 ). We purchased 100,000 additional email addresses of people randomly selected from across the country, including rural areas and excluding the areas covered by the first 350,000 emails. A total of 1,116 responses were received from the email list. Unfortunately, major email service providers quickly began marking our survey invitations as spam, while some smaller providers did not. While we took several steps to mitigate this issue, including changing the wording of the emails, changing the source of the emails (a uic.edu, asu.edu, or covidfuture.org email address), we were ultimately not able to fully solve this problem and saw a lower response rate from individuals with addresses from major email providers.
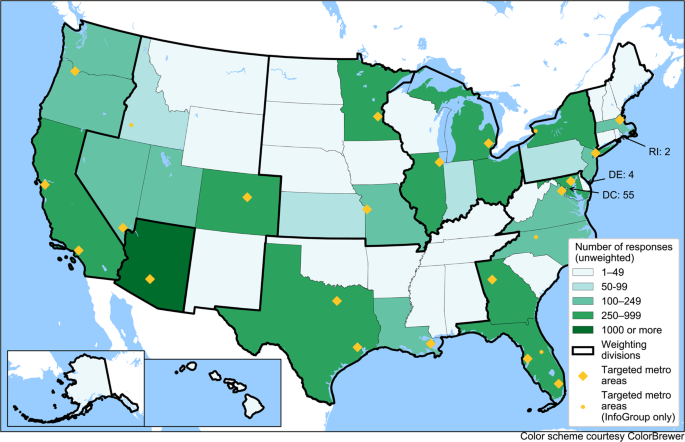
Distribution of survey respondents by the state of residence for survey dataset version 1.0. Alaska and Hawai’i are in the same weighting division as California, Oregon, and Washington.
Survey invitation emails were also sent to an additional list of approximately 39,000 email addresses from the Phoenix metropolitan area purchased for a previous survey effort 22 . This list yielded 782 responses. The survey invitation emails were sent using Amazon Web Services (AWS) and through the Qualtrics platform. Every 20 th respondent who was invited through the purchased email addresses received a $10 incentive as a gift card. Respondents also had the option to donate their survey incentive to a charity. Invitees received two reminders as part of efforts to maximize response rates.
An additional 5,250 responses to the Wave 1B survey were collected through a Qualtrics Online Panel. Qualtrics recruits these respondents from a variety of panels maintained by other firms and uses quota sampling to recruit respondents that are demographically representative of the nation. The Qualtrics quotas were set to collect information from 20 U.S. metropolitan areas, mostly consistent with the metropolitan areas sampled from the purchased email list, as well as the states of Ohio, Utah, North Carolina, upstate New York, and rural areas. In order to obtain samples that would represent the population in each of the selected geographies, quotas were imposed in the Qualtrics online panel subsample to guarantee representation based on income, age, race and ethnicity, and education. We requested all respondents to provide their email addresses in order to recontact them for subsequent survey waves. Since the Qualtrics respondents are professional survey takers, we designated most questions as mandatory, and we included attention check questions, which are shown to improve response quality 23 .
The distribution of responses by geography, as well as the targeted metropolitan areas, are shown in Fig. 1 . Figure 2 shows the distribution of responses by recruitment method, available in the “org” variable in the dataset. The geographical targets were chosen based on geographic and metropolitan area size diversity, as well as the state of the virus spread in May 2020.
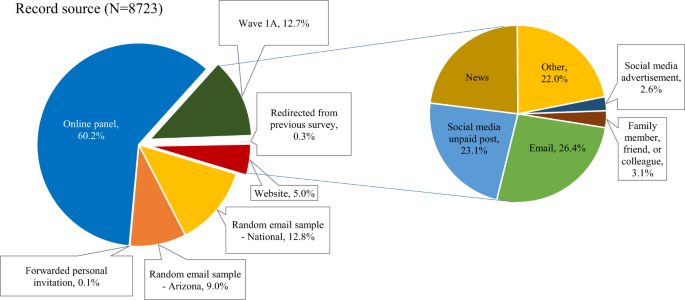
Distribution of Record by Source (from the survey dataset version 1.0).
Figure 1 shows the distribution of survey respondents across the U.S. (50 states and the District of Columbia). Following our recruitment strategy, a greater number of responses come from larger and more urban states. Arizona is overrepresented due to the oversample of Arizona respondents in the email-based deployment. The respondents from the initial Wave 1A sample are also more likely to hail from Arizona as the Arizona State University survey team’s network is heavily Arizona-based. When the data are weighted, any geographic discrepancies at the census division level are controlled and overrepresentation of Arizona is controlled/corrected separately.
Additional survey waves
To monitor how people’s attitudes and behaviors evolve, survey respondents are contacted again with at least two shorter follow-up surveys, approximately four months apart in spring and fall 2021.
Data Records
The survey dataset 24 can be accessed from the ASU Dataverse at: https://doi.org/10.48349/ASU/QO7BTC . The dataset is available in CSV (comma-separated value) format. Since the data will be updated periodically, the data are versioned—in this article, results from the survey dataset version 1.0 are reported. The dataverse also contains the database codebook containing the metadata and explaining the variables. The codebook contains a changelog for each new version.
The respondents to Waves 1A and 1B received similar but not identical surveys. We have merged the responses to these two versions of the survey into the final dataset wherever possible. For some variables, the questions were identical, whereas for other variables, harmonization of similar responses was required. In the dataset, variables ending in ‘_harm’ are harmonized between the two datasets, variables ending in ‘_w1a’ are available only for Wave 1A respondents, variables ending in ‘_w1b’ are available only for respondents from our Qualtrics Online Panel, purchased email lists, and anyone who found the survey via the COVIDFuture web site or email lists after June 19, 2020 (start date of Wave 1B). Variables with no suffix were asked the same way between the two surveys, and no harmonization was necessary. We also provide a file containing only Wave 1B responses and variables, which simplifies analysis of the Wave 1B data.
Technical Validation
Data cleaning.
To monitor respondents’ attention to survey questions in the Qualtrics online panel, attention check questions were included. Respondents were allowed to miss one attention check and be given an opportunity to answer that section again. If they missed an attention check twice, or both attention checks once, their survey was terminated.
We additionally undertook several quality checks to help ensure that the collected data were valid. We removed any respondents who reported that they shop for groceries both in-store and online every day, or expect to after the pandemic, as these are likely to be invalid responses. We also removed respondents who reported strongly agreeing or strongly disagreeing with all COVID-related attitudes, as some of these were worded positively and some negatively. Several additional quality checks were undertaken in the Qualtrics Online Panel as part of Qualtrics’ data cleaning process, including a check for people finishing the survey too quickly.
Respondents that did not report a state of residence, reported living outside the 50 states and the District of Columbia, or did not provide answers to all of the control variables used in the data weighting process described in the next section were removed from the data. Due to this restriction, 558 records with missing control variable information, 59 records with missing home location, and one response from Puerto Rico were not included in the final dataset encompassing responses received through October 14, 2020. Further steps in data preparation will include imputation of missing data, which will allow for some of these omitted records to be recovered in the next version of the dataset. Among the respondents who were not included in the dataset due to missing control variable information, there are 34 respondents who declared their gender as Other; these respondents could not be included because the Census offers no control marginals to weight these records. Further data weighting procedures will attempt to incorporate non-binary gendered individuals on the dataset. Due to the data cleaning and filtering process applied to responses obtained through October 14, 2020, a total of 618 records were not included in the published dataset.
Data weighting
Because the raw data are not fully representative of the U.S. population, weights were calculated using the following control variables: age, education, gender, Hispanic status, household income, presence of children, and number of household vehicles. The weighting procedure accounts for the true population characteristics at the person level. Household-level variables (i.e., income, presence of children, and number of vehicles) were controlled at the person level as well. For example, the marginal distribution used for presence of children refers to the share of adults aged 18 years and older living in household with children, instead of the share of households that have children as it is usually represented. Those marginal distributions were computed using data from the Integrated Public Use Microdata Sample and the American Community Survey (ACS) 2018 1-year data 25 using the sample 18 and older in each of the weighting region boundaries. A noteworthy consequence of this approach is that adjusted household weights are necessary to evaluate household-level characteristics since individuals from larger households are more likely to be represented in the survey (given there are more individuals in these households), and thus have a higher probability of being selected. Weights for household-level analysis can be computed by dividing the person-level weight (provided in the data) by the number of adults in the household.
The national sample was divided into nine regions based on the reported home state (Table 2 ). Each region’s sample was then weighted to match the distributions observed in ACS 2018 1-year estimates 25 , meaning that the survey is demographically representative at the level of each region as well as the entire U.S. The unweighted and weighted survey results are shown in Table 3 ; the weighted results closely replicate population distributions, with inevitable minor deviations on variables that were not controlled in the weighting process.
Weights were calculated using iterative proportional fitting (IPF) procedures embedded within the synthetic population generator PopGen2.0 26 , 27 , 28 . Univariate marginal control distributions were derived from the Integrated Public Use Microdata Sample, American Community Survey (ACS) 2018 1-year data 25 .
Usage Notes
Since the survey will be followed by at least two follow-up survey waves, the database will be updated periodically after the data for each wave is collected, cleaned, and weighted. Each version of the data will be uploaded to the ASU Dataverse and assigned a new DOI number, and all previous versions will remain available to promote reproducibility.
The weights were developed to produce a sample that is representative of the U.S. population, as well as representative of nine divisions within the U.S.: eight census regions (with East and West South Central combined due to small samples in these regions), and a separate category for Arizona due to its large number of respondents. The weights are not guaranteed to produce a representative sample for other (smaller) geographies. When evaluating subsamples at a finer geography (e.g., state or metropolitan area), data users should compare marginal distributions of key demographic variables with the census, and re-weight the data if needed to be representative of the area being analyzed.
Some questions differ between Waves 1A and 1B. Therefore, we have weighted the dataset twice: once including all respondents (Waves 1A and 1B), and once excluding respondents to the Wave 1A sample. Data users should use the Wave 1B weights whenever using variables that are not present in the convenience sample. Since Wave 1A data deviates significantly in terms of population representativeness 4 , there are no weights for questions asked only of Wave 1A respondents. In the file with only Wave 1B responses, only Wave 1B weights are presented.
This unique dataset provides insights on attitudes and behaviors not just before and during pandemic, but also on what might be expected after the pandemic. Possible use cases include modeling of during-pandemic and longer-term changes in mode use, air travel, transit ridership, work from home, and traffic congestion (especially for peak period traffic planning). Published uses of this dataset are documented in Capasso da Silva et al . 29 , Chauhan et al . 30 , Mirtich et al . 31 , and Salon et al . 32 .
Code availability
No codes were developed for this research.
COVID-19 Map. Johns Hopkins Coronavirus Resource Center https://coronavirus.jhu.edu/map.html (2020).
CDC. Coronavirus Disease 2019 (COVID-19). Centers for Disease Control and Prevention https://www.cdc.gov/coronavirus/2019-ncov/prevent-getting-sick/social-distancing.html (2020).
Shamshiripour, A., Rahimi, E., Shabanpour, R. & Mohammadian, A. K. How is COVID-19 reshaping activity-travel behavior? Evidence from a comprehensive survey in Chicago. Transp. Res. Interdiscip. Perspect. 7 , 100216 (2020).
PubMed PubMed Central Google Scholar
Conway, M. W., Salon, D., da Silva, D. C. & Mirtich, L. How will the COVID-19 pandemic affect the future of urban life? Early evidence from highly-educated respondents in the United States. Urban Sci. 4 , 50 (2020).
Article Google Scholar
Hasell, J. et al . A cross-country database of COVID-19 testing. Sci. Data 7 , 1–7 (2020).
Kalkreuth, R. & Kaufmann, P. COVID-19: a survey on public medical imaging data resources. ArXiv Prepr. ArXiv200404569 (2020).
Desvars-Larrive, A. et al . A structured open dataset of government interventions in response to COVID-19. medRxiv (2020).
Cheng, C., Barceló, J., Hartnett, A. S., Kubinec, R. & Messerschmidt, L. Covid-19 government response event dataset (coronanet v. 1.0). Nat. Hum. Behav. 4 , 756–768 (2020).
Zheng, Q. et al . HIT-COVID, a global database tracking public health interventions to COVID-19. Sci. Data 7 , 1–8 (2020).
Xu, B. et al . Epidemiological data from the COVID-19 outbreak, real-time case information. Sci. Data 7 , 1–6 (2020).
Pepe, E. et al . COVID-19 outbreak response, a dataset to assess mobility changes in Italy following national lockdown. Sci. Data 7 , 1–7 (2020).
Killeen, B. D. et al . A County-level dataset for informing the United States’ response to COVID-19. ArXiv Prepr. ArXiv200400756 (2020).
Chen, E., Lerman, K. & Ferrara, E. Tracking social media discourse about the COVID-19 pandemic: Development of a public coronavirus Twitter data set. JMIR Public Health Surveill. 6 , e19273 (2020).
Zarei, K., Farahbakhsh, R., Crespi, N. & Tyson, G. A first Instagram dataset on COVID-19. ArXiv Prepr. ArXiv200412226 (2020).
Alqurashi, S., Alhindi, A. & Alanazi, E. Large arabic twitter dataset on covid-19. ArXiv Prepr. ArXiv200404315 (2020).
Lopez, C. E., Vasu, M. & Gallemore, C. Understanding the perception of COVID-19 policies by mining a multilanguage Twitter dataset. ArXiv Prepr. ArXiv200310359 (2020).
Gensler. US Work from home survey 2020. Gensler-US-Work-From-Home-Survey-2020-Briefing-1.pdf (2020).
Kleinberg, B., van der Vegt, I. & Mozes, M. Measuring emotions in the covid-19 real world worry dataset. ArXiv Prepr. ArXiv200404225 (2020).
Grashuis, J., Skevas, T. & Segovia, M. S. Grocery shopping preferences during the COVID-19 pandemic. Sustainability 12 , 5369 (2020).
Article CAS Google Scholar
Shuja, J., Alanazi, E., Alasmary, W. & Alashaikh, A. Covid-19 open source data sets: A comprehensive survey. Appl. Intell . 1–30 (2020).
Trung, T. et al . Dataset of Vietnamese student’s learning habits during COVID-19. Data Brief 105682 (2020).
Khoeini, S. et al . Attitudes Towards Emerging Mobility Options and Technologies – Phase 2: Pilot and Full Survey Deployment . https://tomnet-utc.engineering.asu.edu/wp-content/uploads/2020/11/TOMNET-Year-2-Project-Report-All-Universities_-Attitudes-Towards-Mobility-Options-Technologies.pdf (2019).
Shamon, H. & Berning, C. Attention check items and instructions in online surveys: Boon or bane for data quality? Surv. Res. Methods Forthcom . (2019).
Salon, D. et al . COVID Future Wave 1 Survey Data v1.0.0. ASU Library Research Data Repository https://doi.org/10.48349/ASU/QO7BTC (2020).
Ruggles, S. et al . IPUMS USA: Version 10.0 Minneapolis, MN: IPUMS https://doi.org/10.18128/D010.V10.0 (2020).
PopGen. MARG - Mobility Analytics Research Group https://www.mobilityanalytics.org/popgen.html (2020).
Ye, X., Konduri, K., Pendyala, R. M., Sana, B. & Waddell, P. A methodology to match distributions of both household and person attributes in the generation of synthetic populations. In 88th Annual Meeting of the Transportation Research Board, Washington, DC (2009).
Konduri, K. C., You, D., Garikapati, V. M. & Pendyala, R. M. Enhanced synthetic population generator that accommodates control variables at multiple geographic resolutions. Transp. Res. Rec. 2563 , 40–50 (2016).
Capasso da Silva, D. et al . How are attitudes toward COVID-19 associated with traveler behavior during the pandemic? Findings https://doi.org/10.32866/001c.24389 (2021).
Chauhan, R. S. et al . COVID-19 related attitudes and risk perceptions across urban, rural, and suburban areas in the United States. Findings https://doi.org/10.32866/001c.23714 (2021).
Mirtich, L. et al . How stable are transport-related attitudes over time? Findings https://doi.org/10.32866/001c.24556 (2021).
Salon, D. et al . The potential stickiness of pandemic-induced behavior changes in the United States. Proceedings of the National Academy of Sciences 118 (27), e2106499118, https://doi.org/10.1073/pnas.2106499118 (2021).
Download references
Acknowledgements
This research was supported in part by the National Science Foundation (NSF) RAPID program under grants no. 2030156 and 2029962 and by the Center for Teaching Old Models New Tricks (TOMNET), a University Transportation Center sponsored by the U.S. Department of Transportation through grant no. 69A3551747116, as well as by the Knowledge Exchange for Resilience at Arizona State University. This COVID-19 Working Group effort was also supported by the NSF-funded Social Science Extreme Events Research (SSEER) network and the CONVERGE facility at the Natural Hazards Center at the University of Colorado Boulder (NSF Award #1841338) and the NSF CAREER award under grant no. 155173. Any opinions, findings, and conclusions or recommendations expressed in this material are those of the authors and do not necessarily reflect the views of the funders.
Author information
Authors and affiliations.
Department of Civil, Materials, and Environmental Engineering, University of Illinois at Chicago, Chicago, IL, USA
Rishabh Singh Chauhan, Ali Shamshiripour, Ehsan Rahimi, Abolfazl (Kouros) Mohammadian & Sybil Derrible
School of Geographical Sciences and Urban Planning, Arizona State University, Tempe, AZ, USA
Matthew Wigginton Bhagat-Conway & Deborah Salon
School of Sustainable Engineering and the Built Environment, Arizona State University, Tempe, AZ, USA
Denise Capasso da Silva, Sara Khoeini & Ram Pendyala
You can also search for this author in PubMed Google Scholar
Contributions
R.P., A.M., S.D., D.S. and S.K. planned the project. D.S., M.C., D.C.S., R.C., E.R. and A.M. prepared the survey questions. M.C., D.C.S. and D.S. designed the survey flow logic. R.C., D.C.S., M.C., D.S. and S.D. deployed the survey. M.C. and D.C.S. performed data cleaning and survey data analysis. D.C.S. weighted the dataset. M.C. and D.S. worked on sending out the incentives to the selected respondents. R.C. prepared the first draft. All the authors made significant contributions to manuscript editing and approving the final version of the manuscript.
Corresponding author
Correspondence to Rishabh Singh Chauhan .
Ethics declarations
Competing interests.
The authors declare no competing interests.
Additional information
Publisher’s note Springer Nature remains neutral with regard to jurisdictional claims in published maps and institutional affiliations.
Rights and permissions
Open Access This article is licensed under a Creative Commons Attribution 4.0 International License, which permits use, sharing, adaptation, distribution and reproduction in any medium or format, as long as you give appropriate credit to the original author(s) and the source, provide a link to the Creative Commons license, and indicate if changes were made. The images or other third party material in this article are included in the article’s Creative Commons license, unless indicated otherwise in a credit line to the material. If material is not included in the article’s Creative Commons license and your intended use is not permitted by statutory regulation or exceeds the permitted use, you will need to obtain permission directly from the copyright holder. To view a copy of this license, visit http://creativecommons.org/licenses/by/4.0/ .
The Creative Commons Public Domain Dedication waiver http://creativecommons.org/publicdomain/zero/1.0/ applies to the metadata files associated with this article.
Reprints and permissions
About this article
Cite this article.
Chauhan, R.S., Bhagat-Conway, M.W., Capasso da Silva, D. et al. A database of travel-related behaviors and attitudes before, during, and after COVID-19 in the United States. Sci Data 8 , 245 (2021). https://doi.org/10.1038/s41597-021-01020-8
Download citation
Received : 14 December 2020
Accepted : 29 July 2021
Published : 23 September 2021
DOI : https://doi.org/10.1038/s41597-021-01020-8
Share this article
Anyone you share the following link with will be able to read this content:
Sorry, a shareable link is not currently available for this article.
Provided by the Springer Nature SharedIt content-sharing initiative
This article is cited by
response willingness in consecutive travel surveys: an investigation based on the national household travel survey using a sample selection model.
- F. Atiyya Shaw
- Kari E. Watkins
Transportation (2023)
Tracking the state and behavior of people in response to COVID-19 through the fusion of multiple longitudinal data streams
- Mohamed Amine Bouzaghrane
- Hassan Obeid
- Joan Walker
Quick links
- Explore articles by subject
- Guide to authors
- Editorial policies
Sign up for the Nature Briefing newsletter — what matters in science, free to your inbox daily.

quality-assurance
Quality Assurance (QA) for Open Travel Data (OPTD)
View the Project on GitHub opentraveldata/quality-assurance
- Quality Assurance (QA) for OpenTravelData (OPTD)
- Table of Content (ToC)
Ingest processors
Through a pre-built docker image, with a manually built docker image, through a local cloned git repository (without docker), pyenv and pipenv, launch the python checkers.
- Re-set the read-write property of indices
Simplified pipeline and index
Por full index and pipeline, optd consistency and geonames id, por having no geo-location in optd, city por not in optd, multi-city por in optd.
- OPTD vs IATA
State codes
Optd vs un/locode, airport bases / hubs, airline networks, airline appearing in schedules but not in optd, example - optd consistency and geonames.
Table of contents generated with markdown-toc
That repository features scripts to check the quality of the data files produced by the Open Travel Data (OPTD) project.
Though it is not well maintained, that project should produce a Quality Assurance (QA) dashboard, much like Geonames’ one . See the Geonames use case on the Data Quality reference page for more details.
For now, the results of the data quality checks are available on the Transport Search data QA page . For instance, for the 2 June 2021 snapshots:
- Results of the validation checks are published on: https://transport-search.org/data/optd/qa/2021-06-02/results/
- Corresponding reference data sets (which have been checked): https://transport-search.org/data/optd/qa/2021-06-02/to_be_checked/
The corresponding checkers are scripts, maintained in a dedicated checkers/ directory of this repository. Most of them are written in Python, but any other programmation language may be used.
And, hopefully, the QA dashboard will be powered by container images generated thanks to that repository as well.
Travis CI builds are partially covering the tests in https://travis-ci.com/opentraveldata/quality-assurance
Most of the scripts generate CSV data files, which can then be uploaded in databases (classical relational database systems (RDBMS) such as PostgreSQL or ElasticSearch (ES)), or served through standard Web applications. For historical reasons, some scripts may still generate JSON structures on the standard output. In the future, JSON should be used only for metadata, not for the data itself.
The CSV reports are published (thanks to Travis CI) to an OPTD-operated ElasticSearch (ES) cluster. The full details on how to setup that ES cluster, on Proxmox LXC containers, are given in a dedicated elasticsearch tutorial .
For convenience, most of the ES examples are demonstrated both on a local single-node installation ( e.g. , on a laptop) and on on the above-mentioned cluster.
- GitHub repository dedicated to Data Quality
- Transport Search data QA page
- Geonames use case on the Data Quality reference page
- Quality Assurance (QA) images on Docker Cloud
- Induction on monitoring with Elasticsearch
- How to set up a Python virtual environment
ElasticSearch (ES)
- EFK (ElasticSearch, Fluentd, Kibana
- Tutorial - Setup an ES cluster on Proxmox LXC containers )
- Elasticsearch geo-point
- Main: https://www.elastic.co/guide/en/elasticsearch/reference/current/ingest-processors.html
- Grok processor
- CSV processor
- Date processor
- Script processor
Quick starter
- Retrieve the Docker image: $ docker pull infrahelpers/optd-qa:latest
- Launch the Docker-powered scripts: $ docker run --rm -it infrahelpers/optd-qa:latest bash [ build@8ce25cc20a10 opentraveldata-qa ( master )] make checkers [ build@8ce25cc20a10 opentraveldata-qa ( master )] exit
Installation
- See the Dockerfile for more details
- Clone the OpenTravelData (OPTD) Quality Assurance (QA) Git repository : $ mkdir -p ~/dev/geo $ git clone https://github.com/opentraveldata/quality-assurance.git ~/dev/geo/opentraveldata-qa $ pushd ~/dev/geo/opentraveldata-qa $ ./mkLocalDir.sh $ popd
Points of Reference (POR)
- results/optd-qa-por-best-not-in-optd.csv , exhibiting the POR manually curated in the file of best known POR but not present in the generated OPTD public file
- results/optd-qa-por-cmp-geo-id.csv , reporting POR having a Geonames ID inconsistent among the curated file of best known POR and the generated OPTD public file
- Note that a CSV file has a single row, it is the header. So, it can be considered as empty. $ pushd ~/dev/geo/opentraveldata-qa $ pipenv run checkers/check-por-geo-id-in-optd.py $ wc -l results/optd-qa-por-best-not-in-optd.csv results/optd-qa-por-cmp-geo-id.csv 11 results/optd-qa-por-best-not-in-optd.csv 1 results/optd-qa-por-cmp-geo-id.csv $ ls -lFh results/optd-qa-por-best-not-in-optd.csv results/optd-qa-por-cmp-geo-id.csv -rw-r--r-- 1 user staff 400B Jan 10 15:54 results/optd-qa-por-best-not-in-optd.csv -rw-r--r-- 1 user staff 60B Jan 10 15:54 results/optd-qa-por-cmp-geo-id.csv $ popd
- results/optd-qa-por-optd-no-geocoord.csv , reporting the POR having no geo-location (geo-coordinates)
- Note that if a CSV file has a single row, it is the header. So, it can be considered as empty. $ pushd ~/dev/geo/opentraveldata-qa $ make results/optd-qa-por-optd-no-geocoord.csv pipenv run python checkers/check-por-optd-no-geocoord.py && \ wc -l results/optd-qa-por-optd-no-geocoord.csv && head -3 results/optd-qa-por-optd-no-geocoord.csv 1 results/optd-qa-por-optd-no-geocoord.csv iata_code^geo_id^loc_type $ popd
- results/optd-qa-por-city-not-in-optd.csv , reporting the POR in the curated file of best known POR with cities not referenced as a city in the generated OPTD public file
- Note that if a CSV file has a single row, it is the header. So, it can be considered as empty. $ pushd ~/dev/geo/opentraveldata-qa $ make results/optd-qa-por-city-not-in-optd.csv pipenv run python checkers/check-por-city-not-in-optd.py && \ wc -l results/optd-qa-por-city-not-in-optd.csv && head -3 results/optd-qa-por-city-not-in-optd.csv 1 results/optd-qa-por-city-not-in-optd.csv iata_code^optd_pk^loc_type^geo_id^city_code $ popd
- results/optd-qa-por-multi-city.csv , reporting POR with multiple cities
- results/optd-qa-por-multi-city-not-std.csv , reporting POR with multiple cities not following the sorting order of PageRank values
- Note that if a CSV file has a single row, it is the header. So, it can be considered as empty. ```bash $ pushd ~/dev/geo/opentraveldata-qa $ make results/optd-qa-por-multi-city.csv pipenv run python checkers/check-por-multiple-cities.py && wc -l results/optd-qa-por-multi-city.csv results/optd-qa-por-multi-city-not-std.csv && head -3 results/optd-qa-por-multi-city.csv results/optd-qa-por-multi-city-not-std.csv 111 results/optd-qa-por-multi-city.csv 30 results/optd-qa-por-multi-city-not-std.csv 141 total ==> results/optd-qa-por-multi-city.csv <== iata_code^optd_pk^loc_type^geo_id^city_code_list^page_rank ADJ^ADJ-A-250437^A^250437^AMM,ADJ^0.09819215728644931,0.0 AGY^AGY-R-10377026^R^10377026^MUC,AGY^0.35785165780444,0.0
==> results/optd-qa-por-multi-city-not-std.csv <== iata_code^optd_pk^loc_type^geo_id^city_code_list^page_rank BQC^BQC-B-11279243^B^11279243^BQC,YQB^0.006501240960634933,0.05835677851287664 BVV^BVV-A-8030061^A^8030061^BVV,ITU^0.0,0.006116247321847354 $ popd
The checker is the same as above ( checkers/check-por-cmp-optd-it.py )
- Check the number of POR having different state codes, broken down by country: $ awk -F '^' '{print $8}' results/optd-qa-state-optd-it-diff.csv|sort|uniq -c |sort -nr |head -5 342 CN 288 FR 185 DE 175 GB 138 RU $
- Check the number of POR having differrent state codes for a specific country: $ awk -F '^' '{if ($8 == "CN") {print $8 "^" $7 "^" $12}}' results/optd-qa-state-optd-it-diff.csv|sort|uniq -c |sort -nr |head -5 33 CN^^GD 24 CN^^NM 24 CN^^HB 19 CN^^ZJ 19 CN^^LN
- Add some exception rules in the optd_state_exceptions.csv CSV file : $ head -3 to_be_checked/optd_state_exceptions.csv pk^state_code^geo_id^source^env_id^date_from^date_to^wrong_state_code^comment AR-C^C^3433955^IR^^^^BA^State code in source does not reflect any of the known standards ( be it ISO 3166-2 or IATA codes ) , but seems to correspond to the phonetic initials. References: https://en.wikipedia.org/wiki/ISO_3166-2:AR, https://en.wikipedia.org/wiki/Provinces_of_Argentina AR-H^H^3861887^IR^^^^CH^State code in source does not reflect any of the known standards ( be it ISO 3166-2 or IATA codes ) , but seems to correspond to the first two letters of the state name. References: https://en.wikipedia.org/wiki/ISO_3166-2:AR, https://en.wikipedia.org/wiki/Provinces_of_Argentina
- results/optd-qa-por-optd-not-in-unlc.csv , exhibiting the POR referenced by OPTD but not by UN/LOCODE
- iresults/optd-qa-por-unlc-not-in-optd.csv , exhibiting the POR referenced by UN/LOCODE but not by OPTD
- Note that if a CSV file has a single row, it is the header. So, it can be considered as empty. $ pushd ~/dev/geo/opentraveldata-qa $ pipenv run checkers/check-por-cmp-optd-unlc.py $ wc -l results/optd-qa-por-unlc-not-in-optd.csv 10349 results/optd-qa-por-unlc-not-in-optd.csv $ ls -lFh results/optd-qa-por- * unlc * .csv -rw-r--r-- 1 user staff 4.7M Dec 13 18:22 results/optd-qa-por-optd-not-in-unlc.csv -rw-r--r-- 1 user staff 763K Dec 13 18:22 results/optd-qa-por-unlc-not-in-optd.csv $ popd
- In order to get the IATA-referenced POR out of UN/LOCODE-referenced ones: $ pushd ~/dev/geo/opentraveldata-qa $ awk -F '^' '{if ($2 != "") {print $0}}' results/optd-qa-por-unlc-not-in-optd.csv | wc -l 21 $ popd
That script checks, for every airline of the optd_airlines.csv file , that the airport bases/hubs are appearing in the optd_airline_por_rcld.csv file .
Note that both files ( optd_airlines.csv and optd_airline_por_rcld.csv ) will be downloaded from the OpenTravelData project and stored within the to_be_checked directory. If those files are too old, they should be removed (a newer version will then be automatically downloaded and stored again).
Note that a CSV file has a single row, it is the header. So, it can be considered as empty.
The following script displays all the missing airport bases/hubs:
If the script does not return anything, then the check (successfully) passes.
- That script performs, for every airline of the optd_airlines.csv file , some basic statistics on their network, modelled as graph (where POR are nodes and flight segments/legs are edges): $ pushd ~/dev/geo/opentraveldata-qa $ pipenv run checkers/check-airline-networks.py $ wc -l results/optd-qa-airline-network-far-nodes.csv 7 results/optd-qa-airline-network-far-nodes.csv $ ls -lFh results/optd-qa-airline- * .csv -rw-r--r-- 1 user staff 8.8K Dec 13 18:47 results/optd-qa-airline-network-far-nodes.csv -rw-r--r-- 1 user staff 34B Dec 13 18:47 results/optd-qa-airline-network-zero-distance.csv -rw-r--r-- 1 user staff 87B Dec 13 18:47 results/optd-qa-airline-network-zero-edges.csv -rw-r--r-- 1 user staff 70B Dec 13 18:47 results/optd-qa-airline-por-not-in-optd.csv -rw-r--r-- 1 user staff 136B Dec 13 18:47 results/optd-qa-airline-zero-coord-por-in-optd.csv $ cut -d '^' -f1 ,1 results/optd-qa-airline-network-far-nodes.csv | grep -v "^airline" 9W B5 KD NS P2 X3 $ cat results/optd-qa-airline-network-zero-edges.csv | grep -v "^airline" BY^MAN^MAN^1.0 MT^BHX^BHX^1.0 ZB^LBA^LBA^1.0 $ popd
- That script checks, for every airline appearing in the optd_airline_por_rcld.csv file , whether they are also referenced by OpenTravelData (OPTD) in the optd_airlines.csv file : $ pushd ~/dev/geo/opentraveldata-qa $ pipenv run check-airline-sched-in-optd.py $ wc -l results/optd-qa-airline-schd-not-in-optd.csv 28 results/optd-qa-airline-schd-not-in-optd.csv $ head -3 results/optd-qa-airline-schd-not-in-optd.csv airline_code 9Y AJA $ popd
Publishing to ElasticSearch (ES)
- Ingest the data: $ export TIMESTP = " $( date -u + '%Y-%m-%d %H:%M:%S' ) " $ tail -n +2 results/optd-qa-por-best-not-in-geo.csv | while IFS = ; read -r -a arr ; do curl -XPOST "http://localhost:9200/optd-qa-por-full-v1/_doc?pipeline=parse_optd_por_full_csv" -H "Content-Type: application/json" -d "{ \" tag \" : [ \" optd \" , \" qa \" , \" checker \" , \" por \" , \" geonames \" , \" check-por-geo-id-in-optd.py \" , \" optd-qa-por-best-not-in-geo.csv \" ], \" timestamp \" : \" ${ TIMESTP } \" , \" optd_qa_por_full \" : \" ${ arr [@] } \" }" ; done % Total % Received % Xferd Average Speed Time Time Time Current Dload Upload Total Spent Left Speed 100 660 100 186 100 474 1430 3646 -- : -- : -- -- : -- : -- -- : -- : -- 5076 % Total % Received % Xferd Average Speed Time Time Time Current Dload Upload Total Spent Left Speed 100 835 100 186 100 649 712 2486 -- : -- : -- -- : -- : -- -- : -- : -- 3199 { " _index " : " optd-qa-por-full-v1 " , " _type " : " _doc " , " _id " : " 8cSoKHEBu3P1TMMtzG4v " , " _version " : 1 , " result " : " created " , " _shards " : { " total " : 1 , " successful " : 1 , " failed " : 0 }, " _seq_no " : 1 , " _primary_term " : 1 } { " _index " : " optd-qa-por-full-v1 " , " _type " : " _doc " , " _id " : " 8sSoKHEBu3P1TMMtzG6f " , " _version " : 1 , " result " : " created " , " _shards " : { " total " : 1 , " successful " : 1 , " failed " : 0 }, " _seq_no " : 2 , " _primary_term " : 1 } $ export TIMESTP = " $( date -u + '%Y-%m-%d %H:%M:%S' ) " $ wc -l results/optd-qa-por-best-not-in-geo.csv 616 results/optd-qa-por-best-not-in-geo.csv $ ssh root@tiproxy8 -f -L9400 :10.30.2.191:9200 sleep 600 $ tail -n +2 results/optd-qa-por-best-not-in-geo.csv | while IFS = ; read -r -a arr ; do curl -XPOST "http://localhost:9400/optd-qa-por-full-v1/_doc?pipeline=parse_optd_por_full_csv" -H "Content-Type: application/json" -d "{ \" tag \" : [ \" optd \" , \" qa \" , \" checker \" , \" por \" , \" geonames \" , \" check-por-geo-id-in-optd.py \" , \" optd-qa-por-best-not-in-geo.csv \" ], \" timestamp \" : \" ${ TIMESTP } \" , \" optd_qa_por_full \" : \" ${ arr [@] } \" }" ; done % Total % Received % Xferd Average Speed Time Time Time Current Dload Upload Total Spent Left Speed 100 15053 100 8583 100 6470 128k 99538 -- :--:-- -- :--:-- -- :--:-- 226k { ... }
Querying ElasticSearch (ES) and Kibana
The ElasticSearch (ES) REST API is also the one to use for Kibana queries.
- Query: optd-qa-kibana-request-por-non-geo-hist-ctry.json query
- Response: optd-qa-kibana-response-por-non-geo-hist-ctry.json query
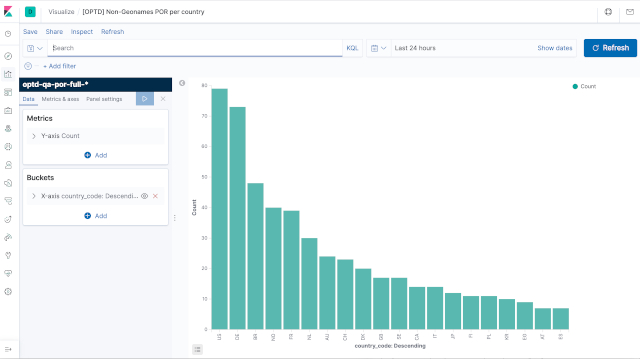
- Launch on the command-line the optd-qa-kibana-response-por-non-geo-map.json query : $ curl -XGET "http://localhost:9200/optd-qa-por-full-v1/_search" \ -H "Content-Type: application/json" \ --data "@elastic/optd-qa-kibana-request-por-non-geo-hist-ctry.json" | jq \ > elastic/optd-qa-kibana-response-por-non-geo-hist-ctry.json $ ssh root@tiproxy8 -f -L9400 :10.30.2.191:9200 sleep 5 ; \ curl -XGET "http://localhost:9400/optd-qa-por-full-v1/_search" \ -H "Content-Type: application/json" \ --data "@elastic/optd-qa-kibana-request-por-non-geo-hist-ctry.json" | jq \ > elastic/optd-qa-kibana-response-por-non-geo-hist-ctry.json
- It generates the optd-qa-kibana-response-por-non-geo-hist-ctry.json response
- Query: optd-qa-kibana-request-por-non-geo-map.json query
- Response: optd-qa-kibana-response-por-non-geo-map.json response
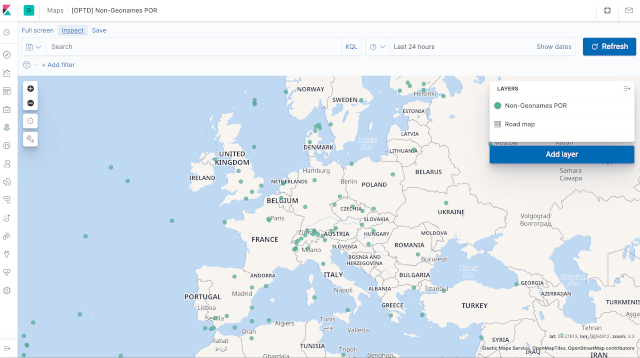
- Launch on the command-line the optd-qa-kibana-response-por-non-geo-map.json query : $ curl -XGET "http://localhost:9200/optd-qa-por-full-v1/_search" \ -H "Content-Type: application/json" \ --data "@elastic/optd-qa-kibana-request-por-non-geo.json" | jq \ > elastic/optd-qa-kibana-response-por-non-geo-map.json $ ssh root@tiproxy8 -f -L9400 :10.30.2.191:9200 sleep 5 ; \ curl -XGET "http://localhost:9400/optd-qa-por-full-v1/_search" \ -H "Content-Type: application/json" \ --data "@elastic/optd-qa-kibana-request-por-non-geo.json" | jq \ > elastic/optd-qa-kibana-response-por-non-geo-map.json
- It generates the optd-qa-kibana-response-por-non-geo-map.json response
- Fares and Tolls
- Access-A-Ride Paratransit
- Accessibility
- About the MTA
- Give feedback
- Sign up for service alerts
- New York City Transit
- Bridges & Tunnels
- Long Island Rail Road
- Metro-North Railroad
- Other agencies and departments
- Planned Service Changes
- Elevator & Escalator Status
- Safety and Security
- Transparency
- Contact the MTA
- Media Relations
- Procurement and solicitations
Open data discoveries for MTA enthusiasts
It’s been a whirlwind month for MTA Open Data! Our team has been busy celebrating NYC Open Data Week , which happens annually in March. We’ve published additional resources, extra blog posts, and released our annual update to the Open Data Plan. To cap off our busy month, we’re back with one more open data-focused blog post that is focused on you, the user, and ideas for topics you can explore using our wide array of open datasets ! We have more data and ideas than time allows our staff to investigate, so we hope this post will help jumpstart your next open data project!
Create a visual
Our first and simplest suggestion is to use our data to create a visual. Our own site, metrics.mta.info , uses Python to create interactive visuals using our open datasets on data.ny.gov . We love a clean line chart, like the ones we publish for Subway Mean Distance Between Failures and Metro-North On-Time Performance . For some of our visuals, like Major Felonies , we offer the option to toggle between line and stacked bar charts.
One dataset we love but haven’t visualized on our site yet is Express Bus Capacity . This data shows how crowded express buses are for each route by hour of the day and direction. If paired with GTFS files for the geographic shape of the bus route, the level of crowding on express bus routes across the city by time of day could be visualized, with the color and/or width of the lines of each route changing depending on the level of crowding.
And remember, visualizations don’t need to be technical or even digital. We’re big fans of the group Data through Design and the multimedia expressions they create using open data.
There are so many great data visualization tools and techniques. Whether you have a tried-and-true tool or are in the process of learning something new, we hope you’ll try using one of our hundreds of MTA open datasets in your next visual!
Explore trends over time
Another great way to make use of MTA data is to explore trends in data over time. Our monthly Bridges & Tunnels dataset goes back to 2005, while the B&T hourly dataset goes to 2010. These datasets could be used to look at the impacts of weather, including restrictions on certain trucks during storms; gas prices; and events, like the UN General Assembly, on traffic.
The MTA Subway Customer Journey-Focused Metrics: 2015-2019 and MTA Subway Customer Journey-Focused Metrics: Beginning 2020 datasets have a column for estimated ridership by individual subway line, not by station, based on subway modeling: see our recent blog post on the subway ridership model for more information. This field allows data explorers to compare ridership by subway line for both peak and off-peak periods over time-information that is not available elsewhere.
There is a lot of analysis that could be done with the Paratransit Ride Time dataset , which has trips by origin and destination borough, peak or off-peak hours, trip distance, and total ride time, among other categories. This dataset could be used to look at the change in average trip distance over time, or the percentage of trips within each trip distance category, the change in and percentage of trips between specific borough combinations, the average speed (based on the midpoint distance for the distance category), or the average travel time between boroughs or different trip distances over time.
Play matchmaker and find relationships
Our Bus and Subway Hourly Ridership datasets were updated earlier this year to add fare class to the dataset, allowing data users to see the type of fare payment riders are using. This data could be used to look at the subway stations and bus routes (and hours) with the highest or lowest percentage of ridership from categories like OMNY, MetroCard Senior/Disability, Students, and Fair Fares. This could provide insight on who is using these stations or routes. This data could also be compared over time, looking at changes in the percentage of senior riders and riders with disabilities who are using OMNY vs. MetroCard.
This data could be joined with data from the American Community Survey to allow users to estimate the percentage of eligible people living in a community around a station or bus route who could but are not taking advantage of reduced-fare programs. It could also be joined with weather data to see how ridership changes when there is rainfall or extreme weather.
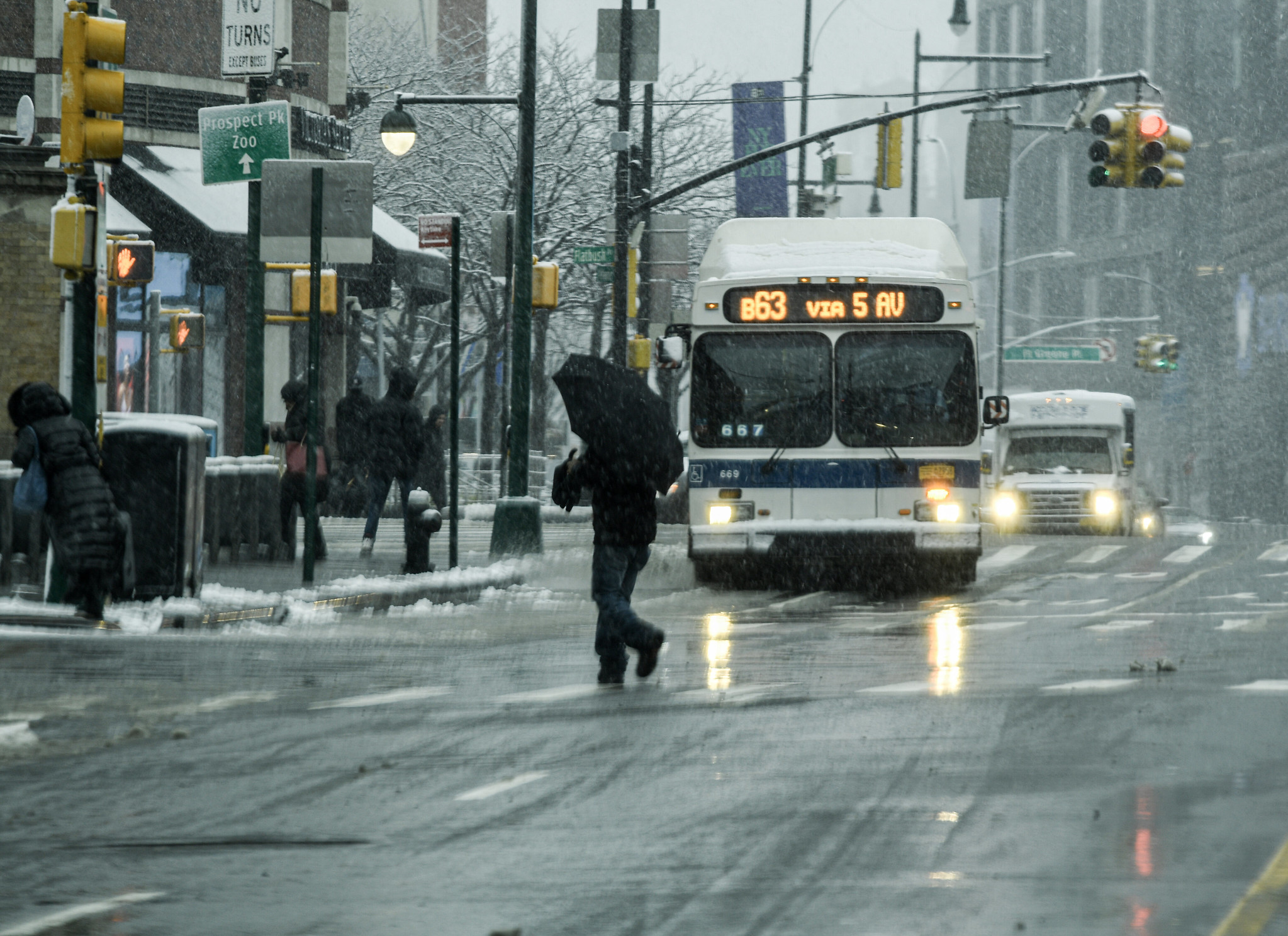
In the last year, our team re-released the paratransit datasets to improve their format and quality. One way data users can use these datasets is to look into the impact of on-time performance (either drop-off or pick-up ) on the number of trips between specific boroughs (available in the Ride Time dataset).
Data users can also take advantage of the coordinate locations of subway stations from the MTA Subway Stations dataset to evaluate the impact proximity to a subway station has on the built environment and the people who live nearby. This dataset could be compared with third-party rent data, income and demographic data from the American Community Survey, or even with street tree health or proximity to an FDNY firehouse .
Try something new
We hope this post has sparked some ideas for interesting analyses you can do using MTA Open Data! If you make something cool using our data, or if you have other ideas for interesting analyses, please share them publicly and let us know by emailing [email protected] .
About the authors
Lisa Mae Fiedler is a manager on the Data & Analytics team, leading the MTA’s Open Data Program.
Shaul Picker is a graduate intern with the Data & Analytics team, specializing in open data.

OFFENER TOURISTISCHER DATENSTANDARD
DAS DATENFORMAT FÜR HÖCHSTE DATENQUALITÄT

EIN FORMAT - EIN VEREIN
LEISTUNGSSTARK. OFFEN. KOSTENFREI

OTDS - DATENSTANDARD DER TOURISMUSBRANCHE
BEI DER MEHRZAHL DER VERANSTALTER IMPLEMENTIERT
A high performance data standard is transforming the travel industry: The Open Travel Data Standard OTDS facilitates travel offers performing on highest data quality levels into the travel sales channels. The modern XML standard accelerates and specifies the production of travel offers while at the same time delivering significantly more booking relevant details and number of data records. The travellers benefit from a comprehensive and meaningful travel offer matching their search terms which in addition can be narrowed down to their individual whishes via filters. Last but not least reliable pricings and availability declarations ease the way to booking conversion.
- The OTDS standard
The OTDS association

OTDS stands for the Open Travel Data Standard – a powerful data format developed from experts within the travel industry to serve the branch. All interested market player may use the format and its extensive documentation free of charge.
The XML-format OTDS contains all master data of the included travel components as well as all necessary product and pricing rules. Travel providers may use OTDS to distribute their offer into all sales channels, whether it be to travel agencies or to travel websites.
The Open Travel Data Standard OTDS is published by the OTDS association (OTDS e.V.) in Berlin, Germany. The members of the association represent almost every branch of the travel industry and join forces to continuously refine and extend the data format OTDS.
Via the committees of the association each member is actively involved in the decision-making process and into the development roadmap and realisation. The joint future development of OTDS and the communication between the members takes places within the exclusive internet-based member’s forum.
OTDS registration
Your Username *
Your Password * (min. 8 Characters)
Password repeat *
First name *
Last name *
Your eMail adress *
Hereby I accept the Privacy Policy
Privacy Policy
The OTDS association commits itself to compliance with the legal regulations for data security, which are regulated in particular in the Federal Data Protection Act (BDSG) and in the Telemedia Act (TMG).
In compliance with the laws BDSG and TMG the OTDS association collects and uses personal data of each user registering for downloading the OTDS standard.
The data entered by the user in this context are utilized by the OTDS association to create and maintain a user profile consisting of user name, a password chosen by the user which is being depicted only encrypted in our database, first and last name, e-mail address, and company. In addition date and time of the first registration and – for future logins – the date and time of the respectively last login is being archived.
By registering you declare your agreement with the OTDS association creating a personalized user profile within the scope of your registration for www.otds.de. You also declare your agreement with anonymized analyses of the entries within our database. Furthermore you declare your agreement with your e-mail address being used for sending you your login-confirmation and for contacting you in urgent matters concerning your OTDS-registration.
Link to external website
If you activate a link on www.otds.de leading to an external website, the external provider might obtain the information from your browser, which of our webpages was your origin before changing to his internet page. For the use of this data as well as for the content on those external websites exclusively the external provider is responsible.
Bitte lasse dieses Feld leer.
" style="background-color: #fff;border-color: #fff;color: #ea5c00;">
News & Events

freshcells joins the OTDS association
Since 2005, the software and consulting company freshcells systems engineering GmbH has been supporting many companies also in the travel industry in […]

Registrierung für den OTDS-Summit (6) geöffnet
Sorry, this entry is only available in German. Der OTDS-Verein freut sich sehr, die OTDS-Summit Reihe wieder aufzunehmen: In der 6. Auflage […]

OTDS honored for 10 years of DRV membership
At this year’s Annual General Assembly of Deutscher Reiseverband DRV in Berlin it became briefly ceremonial for the OTDS association: OTDS Chairman […]
THE OTDS STANDARD
What is the otds format capable of and what makes it so special, how does otds support tour operators and sales channels.
Strengths of OTDS - The data format OTDS is designed to improve the transmission of provider’s product data such as tour operators, airlines, rental car companies, or other supplier to the distribution systems that process those data for the use in travel search tools used in travel agencies or in travel website booking engines.

OTDS significantly minimizes the production time for data exports of tour operators or their Inventory Management Systems. Compared to the standards used to date such as INFX or KATI the production time is reduced by at least 75 percent. In addition incremental updates allow for deliveries of parts of the portfolio with the corresponding reduced file generation time.

Thanks to OTDS tour operators and other provider may decrease the volume of their data delivery to distribution systems significantly: In practice sizes for OTDS-files reach no more than 10% of the size for INFX-deliveries containng the same amount of offers. As with OTDS rather price- and product rules than complete offers are expressed, considerably more offers can be transferred in even smaller files. The effect on transfer- and loading times is correspondingly positive – while at the same time data quality is pushed to a higher level.

Due to the precise definition of price- and product rules by the tour operator in OTDS there is no scope for interpretation when processing the data. As a result the data quality increases – trips are displayed with reliably calculated prices and availabilities within the consulting tools of travel agencies and in the IBEs. Also total prices for families are feasible. In general with OTDS 95% of all prices are identical with those of the booking request. More that 90% of the trips are available and bookable.

Free of charge
OTDS as the open, nonproprietary standard is available free-of-charge and may be used by any tour operator- and distribution system – regardless of the technology deployed (e.g. central caches or local player). This ensures independence from proprietary data standards or -formats for tour operators and distribution systems.

OTDS already allows the transfer of all data for the most relevant travel components accommodation, flight, packaged travel as well as add-on services such as transfers, rental cars, insurances, activities, or parking lots. In addition OTDS contains all product- and price rules necessary for correct price calculation. All relevant information needed for product display, availability check, booking requests and the booking itself are also included.

OTDS is compatible for various systems: it is the powerful standard of choice for providing travel data to central cache systems as well as all filling players located in the sphere of the supplier. OTDS also supports different external attribution systems for describing the travel products such as Giata Facts and DRV GlobalTypes.
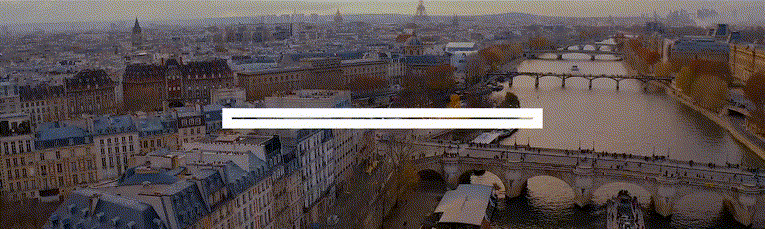
SEE THE OTDS STANDARD FOR YOURSELF
- The value of OTDS
- OTDS versions
The leading distribution systems in the German market already interpret and process OTDS. By doing so, the optimized data are available within their frontoffice tools for travel agencies as well as in their internet booking engines. The tour operator webpages also can be equipped with the enhanced OTDS-data, so that customers searching for travel offers in travel agencies or in the internet can rely on meaningful offers with reliable pricing and availability information and including add-on services. The OTDS format allows for significantly more details, options, room- and flight alternatives.
Families can rely on the total price of a trip when transmitted with OTDS from the very first display on. Price corrections resulting from booking requests are omitted thanks to reliable adult- and children prices and explicit rules for the price calculation in dynamically packaged travel offers.

„BEST TRAVEL TECHNOLOGY SOLUTION 2012“
The travel industry works with a large number of data formats. Those formats help to display the countless travel offers of tour operators and other provider that reach the consumer via various distribution channels. All information about a trip are being processed so that the IT systems within the distribution chain can depict those data. But the travel wishes of the consumer today are much more individual than ten years ago – the travel offers have to meet this change. And also tour operators and other supplier, online portals and travel agencies expect more from the technology they use. Legacy data formats today cannot fulfill those expectations anymore.
So far provider in the travel industry had to translate their travel offers into several data formats in order to supply the various distribution channels. In spite of the many formats the provider often did not succeed in highlighting their specific competitive advantages: the one format lacks a space for submitting taxi transfers, the other format cannot deal with child price reductions or specific child care offers.
So a new, common, open format was needed that delivers all relevant information regarding a travel offer into all distribution channels. This is precisely the intention of OTDS. At the same time it shall reflect the requirements of as many market player as possible and refine itself as an open data format. Thanks to the cost-free download at this website OTDS is freely accessible to all interested travel organisations and thus can establish itself as the future market standard.

OTDS versions - OTDS 1.0 was published by the OTDS association in April 2012. Within this first basic version the travel components accomodation, flight, packaged travel and add-on services such as transfer, rental car, parking, insurances etc. together with all the implicated product- and pricing rules already could be represented.
Version OTDS 1.9.3 provided comprehensive options for flight prioritisation and rules for rounding monetary amounts. Together with the multi-currency option and further diversified possibilities for rental cars and insurances those criteria meet the requirements of many tour operator regarding an efficient and extensive display of their travel products in the distribution systems.
With the main version 2.0, non-bookable content elements such as descriptive texts and media content as well as the ability for multilingualism were integrated. Thanks to the introduction of the Compare construct, product components can be combined much more flexibly under predefined conditions. The Accomodation-, Flight- and AddonWrapper allow the mapping of a sequence of flights and several hotels with rooms, meals and durations - an important basis for round trips in OTDS.
The current version OTDS 2.1 as new features among others offers a revision of the BookingNameSpace, introduces @Class to properties and extends the update option for tags.
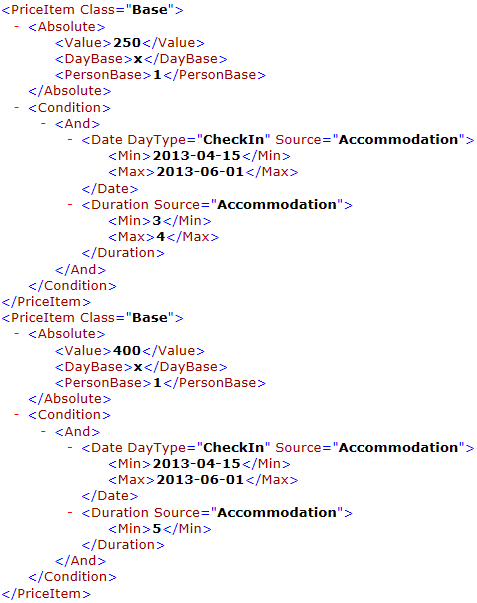
OTDS in Use
A continuously rising number of provider from various areas of the travel industry use OTDS in order to fuel their travel offers to their customers quickly and reliably. Distribution channels and systems can feed on an extensive variety of travel offer data delivered in the up-to-date OTDS format for travel agency tools and Internet Booking Engines:
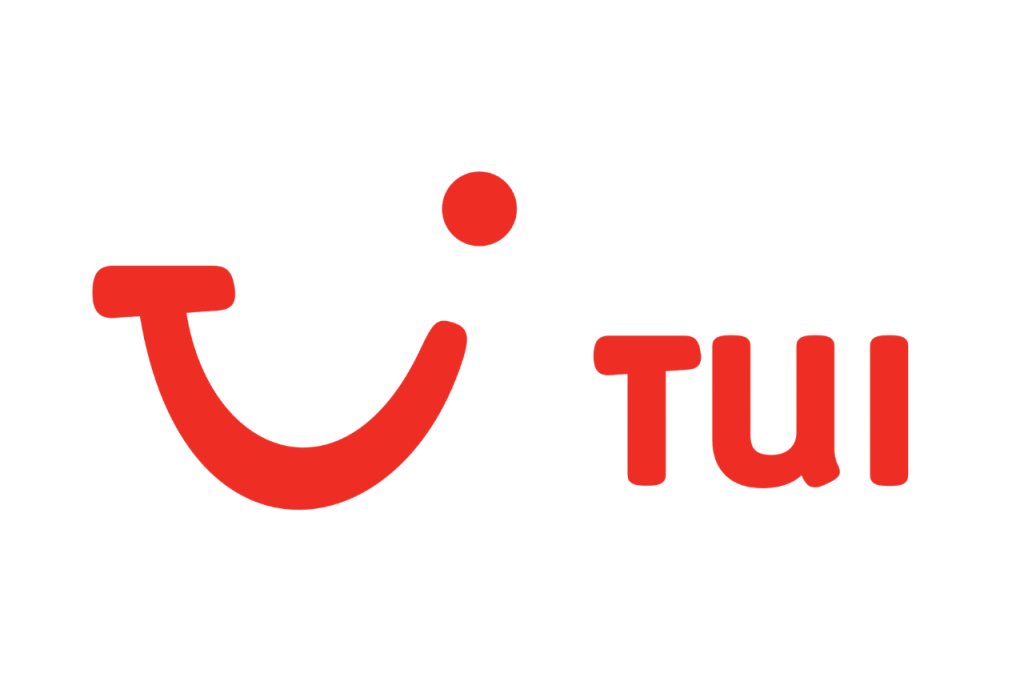
Schmetterling Technology
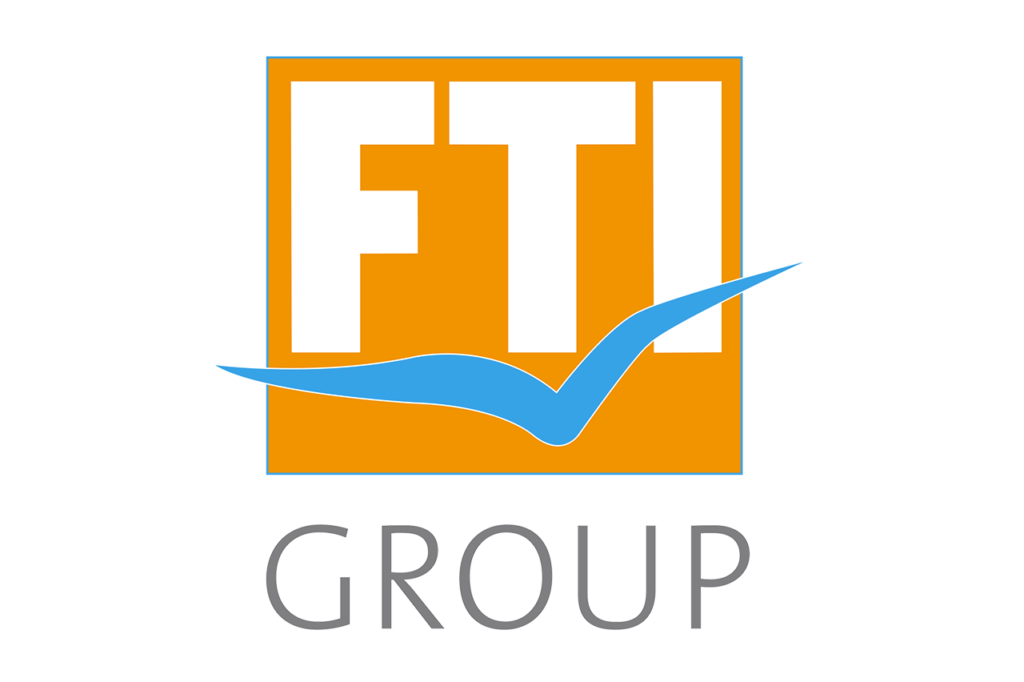
Schauinsland-Reisen

Bentour Reisen

Attika Reisen
Testimonials.
“OTDS: a flexible and living standard, that optimally displays the enormous product diversity of dozens of WBS-tour operators – without converter or third parties. Highest performance in terms of quality and quantity!”

“OTDS is just fun”

“OTDS offers unlimited possibilities for travel offers and overcomes the limitations of other formats.”

Hotel-only, Package travel and flight data in OTDS
Among others the following provider deliver their portfolio of offers in OTDS already or provide it via an OTDS-Player.
"Entire offering" includes the hotel-only, package- and X-offerings of a tour operator brand. The list does not claim to be complete.
- 5 vor Flug (live in Amadeus, Schmetterling and Traffics)
- Aldiana (live in Amadeus, Schmetterling and Traffics)
- Alltours (entire offering live in Amadeus, Schmetterling and Traffics)
- Anex (Hotel-only and packaged trips live in Amadeus, Schmetterling and Traffics)
- anixe (bedbank, Hotel-only data-delivery)
- Attika Reisen (Hotel-only and packaged trips live in Amadeus)
- Bentour Reisen (entire offering live in Amadeus, Schmetterling and Traffics)
- BigXtra (entire offering live in Amadeus, Schmetterling and Traffics, corporate website IBE with OTDS-data)
- Botros Tours (entire offering in the OTDS-Player)
- Bucher Reisen (entire offering live in Amadeus, Schmetterling and Traffics)
- byebye (entire offering live in Amadeus, Schmetterling and Traffics)
- Connected Destination (Hotel-only and packaged trips live in Traffics)
- Coral Travel (entire offering live in Amadeus, Schmetterling and Traffics)
- DERTOUR (entire offering live in Amadeus, Schmetterling and Traffics)
- dta Touristik (Hotel-only and packaged trips live in Amadeus)
- ETI (entire offering live in Amadeus, Schmetterling and Traffics)
- ECCO-Reisen (entire offering live in Amadeus)
- FTI Touristik (entire offering live in Amadeus, Schmetterling and Traffics as well as available in OTDS-Player)
- Gruber Reisen (entire offering in OTDS-Player, live in Amadeus)
- Helvetic Tours (Hotel-only and packaged trips live in Amadeus, Schmetterling and Traffics)
- ITS, ITS Billa und ITS Coop (entire offering live in Amadeus, Schmetterling and Traffics)
- Jahn (entire offering live in Amadeus, Schmetterling and Traffics)
- Kuoni (entire offering live in Amadeus, Schmetterling and Traffics)
- Luxair Tours (entire offering live in Amadeus)
- Meiers Weltreisen (entire offering live in Amadeus, Schmetterling and Traffics)
- Misir (entire offering in OTDS-Player)
- Neckermann Reisen (entire offering live in Amadeus, Schmetterling and Traffics)
- Öger Tours (entire offering live in Amadeus, Schmetterling and Traffics)
- Olimar (entire offering live in Amadeus, Schmetterling and Traffics, OTDS for website)
- Palma (Hotel-only and Packaged trips live in Traffics)
- Phoenix (Hotel-only and Packaged trips live in Amadeus)
- Schauinsland-Reisen (entire offering live in Amadeus, Schmetterling and Traffics, offering available in OTDS-Player)
- Sonnenklar-TV (Hotel-only and packaged trips live in Amadeus and Traffics)
- Travel Partner (Hotel-only also in combination with Addon-Services in various channels)
- TUI (TUI Germany/Switzerland, Airtours DE/CH, XTUI: entire offering live in Amadeus, Schmetterling and Traffics)
- TUI Cruises (offering available in OTDS-Player and on TUI Cruises website)
- Urlaubstouristik (entire offering live in Amadeus)
- vtours (VTOI live in Traffics)
- Windrose Finest Travel (Hotel-only and packaged trips live in Amadeus and Schmetterling)
Flight content in the OTDS format is provided by the travel technology solution providers of Bewotec via its Flex Flight Player (click here for Bewotec airline list ) and Ypsilon.net (click here for Ypsilon airline list ).
OTDS Association
The “Verein zur Förderung eines offenen touristischen Datenstandards e.V.” (Association to promote an Open Travel Data Standard, OTDS e.V. in Berlin, Germany) specifies and develops the data format OTDS for the travel industry. OTDS is based on open, nondiscriminatory, and standardized technologies. The data format is freely available and free-of-charge to everyone, especially to enterprises within the travel industry – regardless of a membership within the OTDS e.V.
OTDS – a modern data standard
It is the objective of the OTDS e.V. to establish its state-of-the-art data format OTDS as a market-wide standard for transmitting all product data relevant for distribution between provider/tour operators and distribution systems. The format is open and non-proprietary. OTDS is independent from any technology concepts and can be used with central caching systems as well as with decentralized player-hub solutions.
Constitution
The OTDS e.V. constitution can be downloaded here.
The constitution defines the objective of the association, explains the membership-status with its privileges and duties, describes the institutions within the organisation as well as their tasks, and lists further regulations.
The OTDS association as of today has 31 members. They represent almost all areas of the travel industry as well as several markets: tour operators, supplier, touristic distribution systems, travel technology supplier, and associations. The OTDS e.V. offers members comprehensive support for their implementation of the data standard as well as the opportunity to actively promote the development of the format by becoming active in the OTDS member forum. Here, the extensive Thematic Specification, which - unlike the Technical Specification - is reserved only for the members of the Association, is also stored.
You may request extensive information on the membership in the form below.
The member companies include:

As an open data standard, OTDS advances the entire travel industry and is not limited to the members of the OTDS association. Companies that want to support this common approach for a powerful, flexible travel data standard can now also do so as promoters of the OTDS association.
You may request extensive information on becoming a promoter in the form below.
The Board of Directors
The executive committee is the leading organ of OTDS e.V. and is elected by the members for three years. In the election in June 2023 Ömer Karaca (Schmetterling) was elected Chairman of the Board. Vice Chairmen are Michael Becher (Bewotec) and Deniz Ugur (Bentour). Michael Fischerkeller (vtours), Haiko Gerdes (TraSo), Thorsten Hendricks (Schauinsland-Reisen), and Lothar Schmitz (Amadeus Leisure IT) complete the Board of Directors.
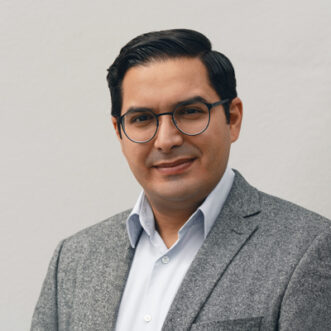
OTDS Association – 8 good reasons for membership
- Active participation in the further development of OTDS
- Personal contacts for support and questions
- Exclusive access to the extensive Thematic specification
- Introduce your own requirements
- Influence on the development of the association
- Information and exchange in the OTDS member forum
- Image effects and public impact
- The ideal factor
Driving efficiency: Leveraging open data for traffic control management
Sharing insights, improving flow, and shaping future mobility
In today's busy cities, managing traffic flow is a constant challenge, but advancements in technology offer promising solutions. Open data is emerging as a valuable tool in this endeavour. By strategically utilising open data, traffic managers can not only alleviate immediate congestion but also make long-term infrastructure improvements.
Analysis of travel times and other factors, such as route reliability , traffic conditions and alternate routes, helps drivers make informed decisions about when and where to travel. Real-time data alerts drivers to potential delays due to congestion or accidents, improving overall traffic efficiency. Moreover, open data enables demand-based strategies for managing traffic congestion over the medium term to be developed. By identifying root causes, such as distribution centre traffic, authorities can implement targeted solutions. For instance, adjusting toll rates or public transit fares based on real-time traffic conditions can help regulate demand and ease congestion.
Data.europa.eu provides access to datasets crucial for effective traffic management. The NRA Traffic Data offers detailed insights collected from traffic counters on the National Road Network, aiding in traffic volume analysis. Additionally, Mobiris provides real-time and historical traffic data for the Brussels region, empowering authorities to make informed decisions about traffic flow and infrastructure investments.
In conclusion, open data offers a wealth of opportunities for enhancing traffic control management. By leveraging insights from open data and utilising available datasets, authorities can implement effective strategies to reduce congestion, improve travel times, and ultimately create more efficient and sustainable transportation systems.
For more news and events, follow us on Twitter , Facebook and LinkedIn , or subscribe to our newsletter .
Text of this article
- View Record
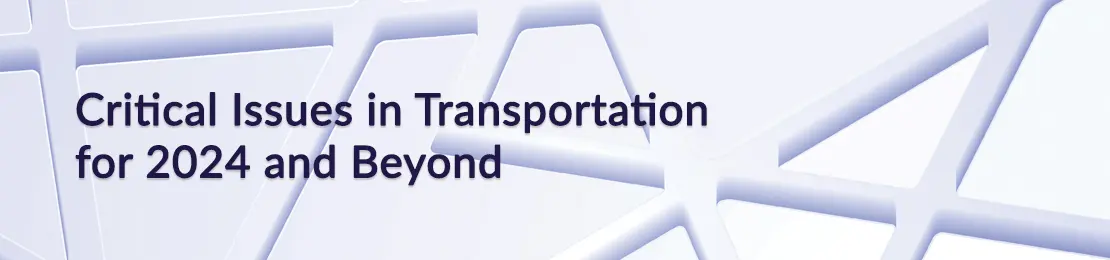
TRID the TRIS and ITRD database
Evaluating the OTAP Interface
The Open Travel data Access Protocol (OTAP) project makes the traffic data from European Traffic Information Centers available to Service Providers through the Internet using standard technology and common formats. It also looks to facilitate the organizational problems encountered in accessing this data, by making procurement information available on a website (www.itsproj.com/otap). This paper details the results of the evaluation of the OTAP interface, both in terms of robustness of the interface for data transfer, and ease of organizational and institutional issues.
ITS America
- Harris, Richard
- Ilgaz, Rana
- Kaltwasser, Joseph
- Visser, Caroline
- 12th World Congress on Intelligent Transport Systems
- Location: San Francisco California, United States
- Date: 2005-11-6 to 2005-11-10
- Publication Date: 2005
- Media Type: Print
- Features: CD-ROM; Figures;
- Pagination: 7p
- Monograph Title: Proceedings of the 12th World Congress on Intelligent Transport Systems
Subject/Index Terms
- TRT Terms: Access control (Communications) ; Data collection ; Information dissemination ; Information systems ; Internet ; Openings ; Standards ; Traffic ; Traffic data ; Travel
- Subject Areas: Data and Information Technology; Highways; Operations and Traffic Management; Planning and Forecasting; I72: Traffic and Transport Planning;
Filing Info
- Accession Number: 01016230
- Record Type: Publication
- Files: TRIS
- Created Date: Jan 31 2006 9:56AM
Cookie Notice
This site uses cookies for performance, analytics, personalization and advertising purposes.
For more information about how we use cookies please see our Cookie Policy .
Cookie Policy | Privacy Policy
Manage Consent Preferences
Essential/Strictly Necessary Cookies
These cookies are essential in order to enable you to move around the website and use its features, such as accessing secure areas of the website.
Analytical/ Performance Cookies
These are analytics cookies that allow us to collect information about how visitors use a website, for instance which pages visitors go to most often, and if they get error messages from web pages. This helps us to improve the way the website works and allows us to test different ideas on the site.
Functional/ Preference Cookies
These cookies allow our website to properly function and in particular will allow you to use its more personal features.
Targeting/ Advertising Cookies
These cookies are used by third parties to build a profile of your interests and show you relevant adverts on other sites. You should check the relevant third party website for more information and how to opt out, as described below.
Announcing Dell Data Lakehouse Analytics Engine powered by Starburst Read the announcement >

- Starburst vs OSS Trino
By Use Cases
- Modern Data Lake
- Artificial Intelligence
- ELT Data Processing
- Data Applications
- Data Migrations
- Data Products
By Industry
- Financial Services
- Healthcare & Life Sciences
- Retail & CPG
- All Industries
- Meet our Customers
- Customer Experience
- Starburst Data Rebels
- Documentation
- Technical overview
- Starburst Galaxy
- Starburst Enterprise
- Upcoming Events
- Data Universe
- Data Fundamentals
- Starburst Academy
- Become a Partner
- Partner Login
- Security & Trust

Fully managed in the cloud
Self-managed anywhere
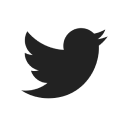
Sr. Manager, Content
The difference between Hudi and Iceberg
Hudi and iceberg are apache software foundation open-source projects that improve the performance of big data architectures, last updated: april 1, 2024, related posts, the icehouse manifesto: building an open lakehouse, tutorial: connecting starburst galaxy to tabular, tutorial: using apache iceberg and trino for data warehousing, building an open lakehouse just got easier with starburst galaxy & tabular.
These open table formats address the issues companies increasingly experience with their legacy platforms running Hadoop and Hive. This article will discuss the differences between Hudi and Iceberg and explain how Iceberg is becoming the cornerstone for modern data lakehouse analytics.
Apache Hudi
The Apache Hudi project got its start in 2016 at Uber. The ridesharing company had built a data lake on Hadoop and Hive, but the batch processing pipelines took hours to complete. Traditional streaming stacks excelled at processing row-based data but could not handle the lake’s columnar data. Uber’s solution became Hudi, an incremental processing stack that reduces ingestion latency from hours to minutes.
Apachi Iceberg
Around the same time Uber was struggling with Hive, Netflix faced a different set of issues. Hive did not handle changes well, so the streaming service needed a new table format that supported ACID (atomicity, consistency, isolation, durability) transactions. Since becoming an open-source project, Apache Iceberg tables are increasingly the preferred choice for data lakes thanks to benefits like:
- Scalability and performance
- ACID transactions
- Schema evolution
- Time travel
Iceberg also provides significant optionality. It supports open file formats like Avro, ORC, and Parquet. The table format also lets users simultaneously use different query engines, such as Flink, Apache Spark, and Trino.
Open table formats: Iceberg, Hudi, Delta Lake
Modern open table formats are essential to maximizing the potential of data lakes by supporting a data warehouse’s processing and analytics capabilities on commodity-priced cloud object storage. Organizations can use Iceberg and Hudi with any Hadoop or other distributed file systems. Another open table format, Delta Lake, is also an option but tends to be used within Databricks platforms.
How does Apache Iceberg handle schema evolution compared to Apache Hudi?
Shifting business priorities and a dynamic data environment frequently require changes to table schema. Older formats like Apache Hive impose significant performance penalties by rewriting entire files in ways that impact existing queries. Schema evolution is one of the key features enabled by modern table formats.
Hudi tables, depending on their configurations, use one of two approaches to schema evolution. Copy On Write (COW) uses columnar formats like Parquet files to store data and performs updates by rewriting the file to a new version. COW is the default approach, proven at scale with high-performance query engines like Trino.
Hudi’s experimental Merge on Read (MOR) approach combines columnar data files with row-based files like Avro to log changes for later compaction. MOR provides greater flexibility, especially for changes to nested columns.
Iceberg uses in-place schema evolution to add, remove, rename, update, and reorder columns without table rewrites. Data files don’t have to be touched because changes are recorded within the table’s metadata. In effect, Iceberg provides a transaction log for each table that includes snapshots of the included data files, statistics to improve query performance, and any changes from previous versions.
Iceberg tables and time travel
Iceberg’s metadata-based approach to documenting change enables time travel , the ability for queries to access historical data. Every change results in a new snapshot that captures the current table’s state, but Iceberg tables keep their old snapshots. Queries can access the table’s list of snapshots to return results from older versions. Rollbacks are common use cases for Iceberg’s time travel functionality, allowing a table to be restored to a previous state after a mistaken change.
What table format should data engineers choose for my data lake?
Each table format brings its own advantages and disadvantages, which data engineering teams need to factor into designing their data architectures. Hudi’s origins as a solution to Uber’s data ingestion challenges make it a good choice when you need to optimize data processing pipelines. In contrast, Netflix developed Iceberg to simplify the big data management issues of the Hadoop and Hive ecosystem. As such, migrating to Iceberg tables is ideal for storing large datasets in a data lake.
Iceberg and Trino MPP SQL query engine, Apache Spark
As mentioned earlier, Iceberg lets different query engines access tables concurrently, allowing data teams to use the most appropriate engine for the job. Trino, a fork of Presto, is a massively parallel processing SQL query engine that uses connectors to query large datasets distributed across different sources.
Trino’s Iceberg connector provides full access to Iceberg tables by simply configuring access to a catalog like the Hive Metastore, AWS Glue, a JDBC catalog, or a REST catalog. Trino will connect to Azure Storage, Google Cloud Storage, Amazon S3, or legacy Hadoop platforms.
Amazon S3, AWS and Iceberg
AWS services like Athena, EMR, and Glue support Iceberg tables to various degrees. Athena, for example, requires Iceberg tables to store data in Parquet files and will only work with Glue catalogs.
What is Iceberg in Snowflake?
Snowflake is a proprietary cloud-based data warehouse solution. Recently, the company began developing support for Iceberg, now in public preview. Snowflake’s users can configure the system to be Iceberg’s metadata catalog or use Snowflake to pull snapshots from either a Glue catalog or directly from an object store.
Getting the most out of Snowflake’s implementation, however, requires integrating Iceberg’s metadata into Snowflake at the risk of greater vendor lock-in.
Start building your open data lakehouse powered by Iceberg table formats
Starburst Galaxy is a modern data lakehouse analytics platform founded by the creators of Trino. With features like federation, near-real-time ingestion, accelerated SQL analytics, and more than fifty connectors, Galaxy unifies enterprise data within a single point of access. Big data becomes easier to manage across a globally distributed architecture, improving compliance with GDPR and other data regulations. At the same time, Galaxy makes data more accessible since data consumers can use ANSI-standard SQL or business intelligence tools to query data anywhere in the organization.
Performance of a data warehouse
Starburst builds upon Trino’s massively parallel processing query engine to give data lakehouses the analytics performance of proprietary data warehouses.
A cost-based optimizer takes SQL queries and evaluates the performance and cost implications of different execution plans, choosing the ideal option to meet data teams’ business criteria.
Starburst’s Cached Views create snapshots of frequently-requested query results to reduce costs and apparent latency. From the user’s perspective, the materialized views are indistinguishable from a fresh query run. And with incremental updates, the cached data remains current.
Additional performance features like pushdown queries and dynamic filtering complete queries faster while also reducing network traffic.
Scale of a data lake
Starburst Galaxy fully enables the scalability enterprises need from their data architectures. A data lake’s object storage provides a central repository for ad hoc, interactive, and advanced analytics. However, it can never hold all the data relevant to insight generation.
By federating data lakes and other data sources within a unified access layer, Starburst Galaxy turns the organization’s entire architecture into a distributed data lakehouse.
Starburst Gravity is the platform’s universal discovery, governance, and sharing layer. Gravity’s automatic cataloging system consolidates metadata from every source, turning Starburst into a central access hub across clouds, regions, and sources.
Gravity provides role-based and attribute-based access controls to streamline governance and support fine-grained access policies down the row and column levels.
The advantages of combining the strengths of Starburst’s analytics platform with the benefits of the Iceberg table format are so strong that Iceberg is the default table format when creating tables in Starburst Galaxy.
A single point of access to all your data
Stay in the know - sign up for our newsletter.
- Resource Library
- Events and Webinars
- Open-source Trino
Quick Links
Get in touch.
- Customer Support
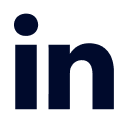
© Starburst Data, Inc. Starburst and Starburst Data are registered trademarks of Starburst Data, Inc. All rights reserved. Presto®, the Presto logo, Delta Lake, and the Delta Lake logo are trademarks of LF Projects, LLC
Privacy Policy | Legal Terms | Cookie Notice
Start Free with Starburst Galaxy
Up to $500 in usage credits included
- Query your data lake fast with Starburst's best-in-class MPP SQL query engine
- Get up and running in less than 5 minutes
For more deployment options:
Please fill in all required fields and ensure you are using a valid email address.
By clicking Create Account , you agree to Starburst Galaxy's terms of service and privacy policy .
Search code, repositories, users, issues, pull requests...
Provide feedback.
We read every piece of feedback, and take your input very seriously.
Saved searches
Use saved searches to filter your results more quickly.
To see all available qualifiers, see our documentation .
- Notifications
Python wrapper around OpenTravelData (OPTD)
opentraveldata/python-opentraveldata
Folders and files, repository files navigation, opentraveldata (optd) data wrapper - python bindings.
Python wrapper around OpenTravelData (OPTD) data sets, for instance to be used by Python software needing to access OPTD data.
- PyPi artifacts: https://pypi.org/project/opentraveldata/
- Source code on GitHub: https://github.com/opentraveldata/opentraveldata
- Docker Cloud repository: https://cloud.docker.com/u/opentraveldata/repository/docker/opentraveldata/quality-assurance
- This Python-wrapper Git repository: https://github.com/opentraveldata/python-opentraveldata
- POR (Points of Reference)): https://www2.transport-search.org/data/optd/por/
- CI/CD deliveries: https://www2.transport-search.org/data/optd/cicd/
- Sourcce code on GitHub: https://github.com/opentraveldata/quality-assurance
- Quality Assurance (QA) reports: https://www2.transport-search.org/data/optd/qa/
- How-to install Python virtual environment with pyenv and pipenv
- How to package modules for Python
- PyPi Travis CI provider
- Launch a Python interpreter:
- Import the opentraveldata library:
- With the default local directory for the data files, that is /tmp/opentraveldata
- If you do not have access rights for writing into that directory, initialize the OpenTravelData object with a directory on which you have writing access rights:
- Display some information about the OpenTravelData object:
- For the main (IATA/ICAO) POR (points of reference) data file:
- For the UN/LOCODE POR (points of reference) data file:
- Download the latest data files (to be done once in a while; it takes a few seconds, depending on the network bandwidth):
- Trigger an exception if the data files have not been properly downloaded:
- Check that the data files have been properly downloaded, and that their sizes are as expected (40 to 50 MB for the IATA/ICAO data file and 4 to 5 MB for the UN/LOCODE data file):
- IATA/ICAO data file:
- UN/LOCODE data file:
- Display the first few lines (here, 3 lines) of the data files:
- Parse the data files and load their content into internal Python dictionaries:
- Retrieve the details for the IEV code:
Installation - configuration
Reference: How-to install Python virtual environment with pyenv and pipenv : https://github.com/machine-learning-helpers/induction-python/tree/master/installation/virtual-env
Install Pyenv, Python 3.9.6, pip and pipenv :
- Clone the Git repository and install the Python virtual environment (with pipenv ):
PyPi credentials for Travis deployment
- Encrypt the PyPi API token with the Travis command-line utility, which stores the encrypted secret token. As the project is managed by travis-ci.com , the --com option has to be added in the command-line:
Package and release the Python module
- Launch the setup.py script:
- Upload/release the Python packages onto the PyPi test repository :
- Upload/release the Python packages onto the PyPi repository :
Test the Python module
- Launch the test:
Contributors 4
- Python 96.4%
- International
7.4 magnitude quake hits Taiwan, strongest in 25 years
By Nectar Gan , Wayne Chang , Jerome Taylor, Antoinette Radford, Deva Lee and Maureen Chowdhury , CNN
Our live coverage of the Taiwan earthquake has moved here.
Search and rescue efforts continue after 7.4 magnitude earthquake rocks Taiwan. Here's the latest
From CNN staff
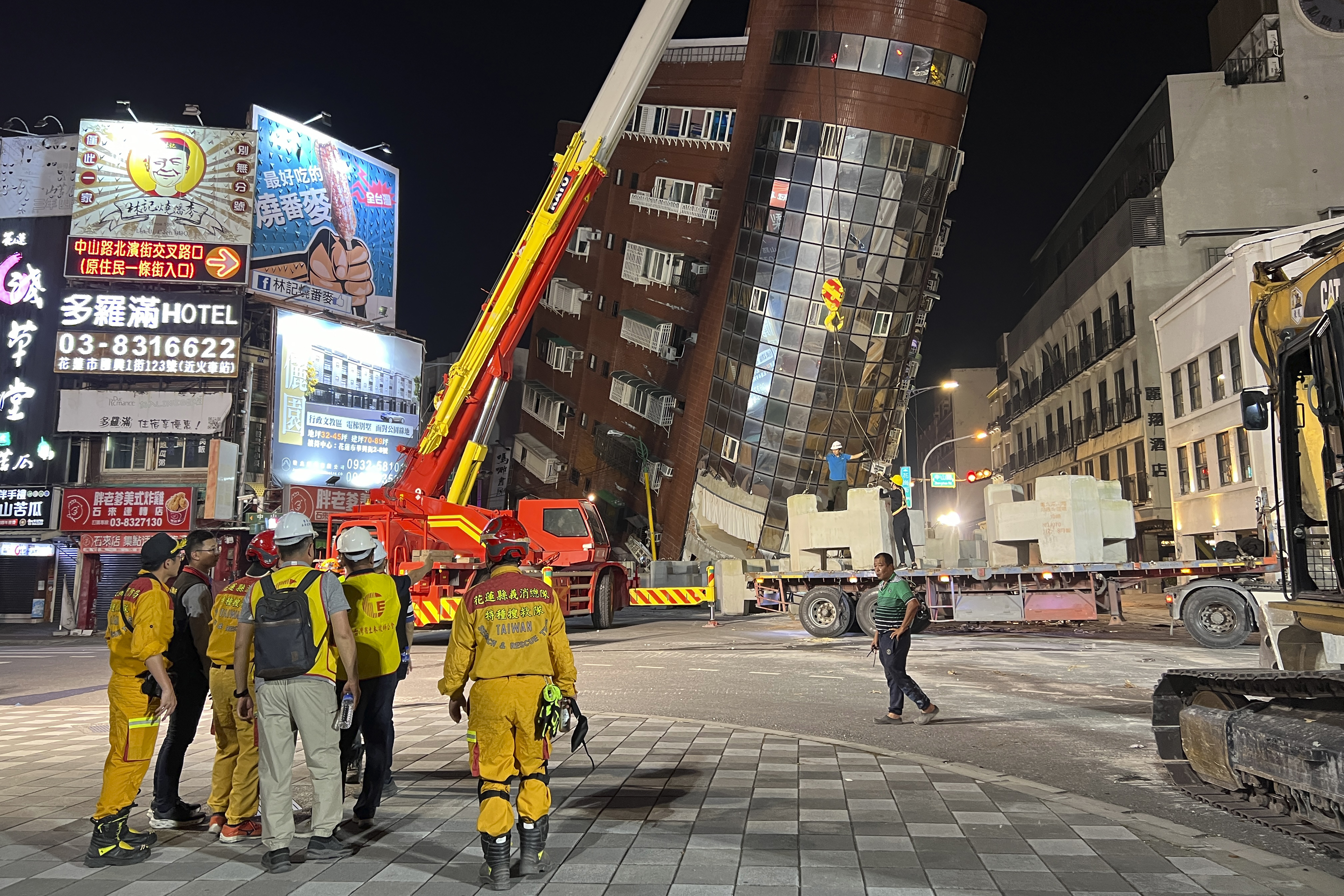
Rescuers are working to free dozens trapped after a 7.4 magnitude earthquake struck the east coast of Taiwan — causing landslides and collapsed structures.
At least nine people have died , more than 900 others are injured and over 100 buildings have been damaged.
The quake is the strongest to hit Taiwan in 25 years .
Here's what else we know:
- The quake: The earthquake hit at 7:58 a.m. local time, 18 kilometers (11 miles) south of the city of Hualien at a depth of 34.8 kilometers, according to the US Geological Survey.
- Aftershocks : The quake was followed by 29 aftershocks greater than a magnitude of 4.0 near the epicenter of the earthquake in east Taiwan so far, according to the United States Geological Survey (USGS). Tremors have been felt across the island, including in Taipei. Tremors as high as magnitude 7 are expected in the following days.
- Hualien County: The region where the quake struck, Hualien County, has a population of about 300,000, around 100,000 of whom live in the main city of Hualien. But many in the region live in remote coastal or mountain communities that can be hard to reach , so it might take time to understand the extent of Wednesday's quake.
- Trapped miners: Taiwan's national fire agency said that 71 people are trapped in two mines in Hualien.
- Power cuts : More than 91,000 households were without electricity, according to Taiwan's Central Emergency Command Center.
- Medical facilities: Hospitals across Taiwan’s capital , Taipei City, are operating normally despite being damaged by Wednesday’s earthquake, according to the Municipal Government.
- US monitoring: The Biden administration is monitoring the earthquake in Taiwan overnight and is prepared to offer assistance, a National Security Council spokesperson said Wednesday.
71 miners trapped in 2 mines in Taiwan after earthquake, national fire agency says
From CNN's Shawn Deng
Taiwan's national fire agency said that 71 people are trapped in two mines in Hualien after a powerful earthquake struck the island.
In the Heping mine, there are 64 people trapped, and seven more are trapped in the Zhonghe mine, the fire agency said in a news conference on Wednesday.
Video shows man swimming in a rooftop pool when massive earthquake hit
When a magnitude of 7.4 earthquake rocked Taiwan on Wednesday, it struck during the morning commute.
Video shows highway roads shaking and even a man being heavily swayed and rocked on a rooftop pool.
Watch the moment here:
Taiwanese semiconductor facilities will resume production overnight following earthquake
Taiwan Semiconductor Manufacturing Company Limited (TSMC), the chipmaking giant, said on Wednesday that its facilities which were impacted by the 7.4 magnitude earthquake are expected to resume production overnight.
TSMC reported that their overall tool recovery is at more than 70% within 10 hours of the earthquake striking the island. Safety systems are also operating normally, TSMC added.
The company noted that a small number of tools were damaged but that there was no damage to its extreme ultraviolet lithography (EUV) tools. Work at construction sites will resume after further inspections are complete, TSMC said.
Earlier, a TSMC spokesperson told CNN they had evacuated some manufacturing plants. All personnel are now safe, TSMC said in an update.
Biden administration monitoring Taiwan earthquake, White House says
From CNN's Sam Fossum
The Biden administration is monitoring the earthquake in Taiwan overnight and is prepared to offer assistance, a National Security Council spokesperson said Wednesday.
"We are monitoring reports of the earthquake impacting Taiwan and continue to monitor its potential impact on Japan. The United States stands ready to provide any necessary assistance. All those affected are in our prayers," a statement from National Security Council spokesperson Adrienne Watson said.
7 major earthquakes have hit Taiwan over the last 50 years
The 7.4 magnitude earthquake that killed at least nine people and injured hundreds Wednesday, is the strongest to hit Taiwan in 25 years .
Over the last 50 years, the island has experienced a total of seven major earthquakes, the last being a 7.1 magnitude quake in 2006 in Pingtung County in southern Taiwan.
The island sits on the Pacific Ring of Fire , which makes it prone to earthquakes.
See a full list of the earthquakes that have hit Taiwan:
29 aftershocks above 4.0 magnitude have occurred near epicenter since earthquake, US Geological Survey says
From CNN's Sara Tonks
There have been 29 aftershocks greater than a magnitude of 4.0 near the epicenter of the earthquake in east Taiwan so far, according to the United States Geological Survey (USGS).
Of these aftershocks:
- One was above 6.0
- 13 have been at or above 5.0
- 14 have been above 4.0.
Forecast during recovery efforts: Tonight's forecast in Hualien City, near the epicenter, calls for increasing cloud coverage. Thursday is looking at mostly cloudy skies with afternoon showers and rain Thursday night and Friday during the day.
Rainfall totals should be relatively light for Taiwan, with models calling for under 25 mm (less than 1 inch) by Friday evening local time.
Watch landslide engulf road after 7.4 magnitude earthquake hits Taiwan
A dashcam camera has caught the moment a large landslide came down a mountain in Taiwan, triggered by a 7.4 magnitude earthquake on Wednesday morning.
The quake is the strongest to have rattled the island in 25 years, killing at least nine people and leaving more than 150 trapped.
Please enable JavaScript for a better experience.
Baltimore Port: What impact will bridge collapse have on shipping?
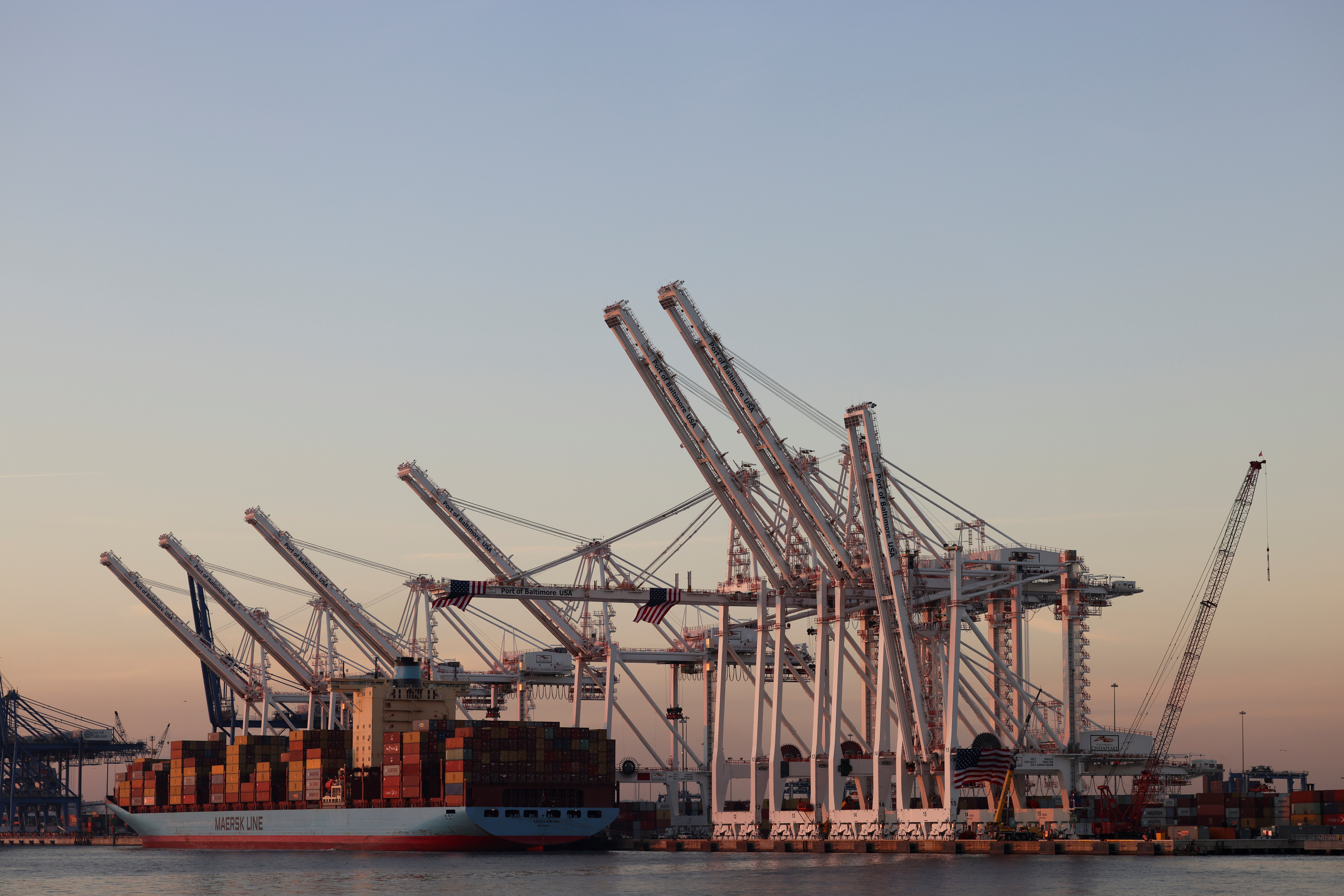
PORT FEATURES
Current status of cargo ships inside port, cruise ships, london metal exchange warehouses, bunker fuel.
Get weekly news and analysis on the U.S. elections and how it matters to the world with the newsletter On the Campaign Trail. Sign up here.
Reporting by Josephine Mason, Nick Carey, Helen Reid, Jonathan Saul, Nigel Hunt, Marwa Rashad, Scott DiSavino, Shariq Khan and Eric Onstad; Compiled by Josephine Mason and Nina Chestney; Editing by Nick Macfie and Nick Zieminski
Our Standards: The Thomson Reuters Trust Principles. , opens new tab
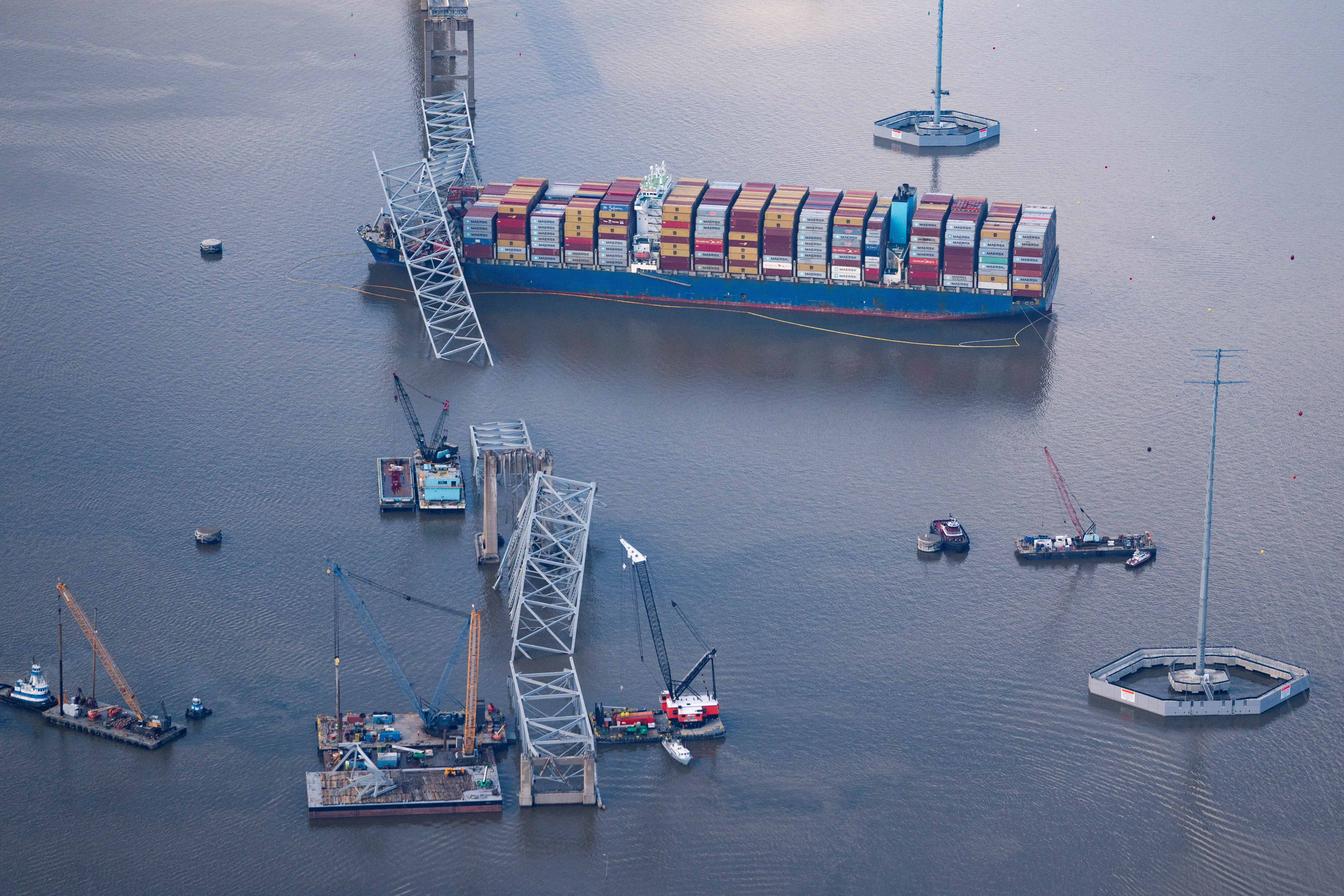
Indian consumer confidence at more than four-year high, cenbank data shows
Consumer confidence in India is at its highest level since mid-2019, a survey conducted by the Reserve Bank of India showed on Friday.
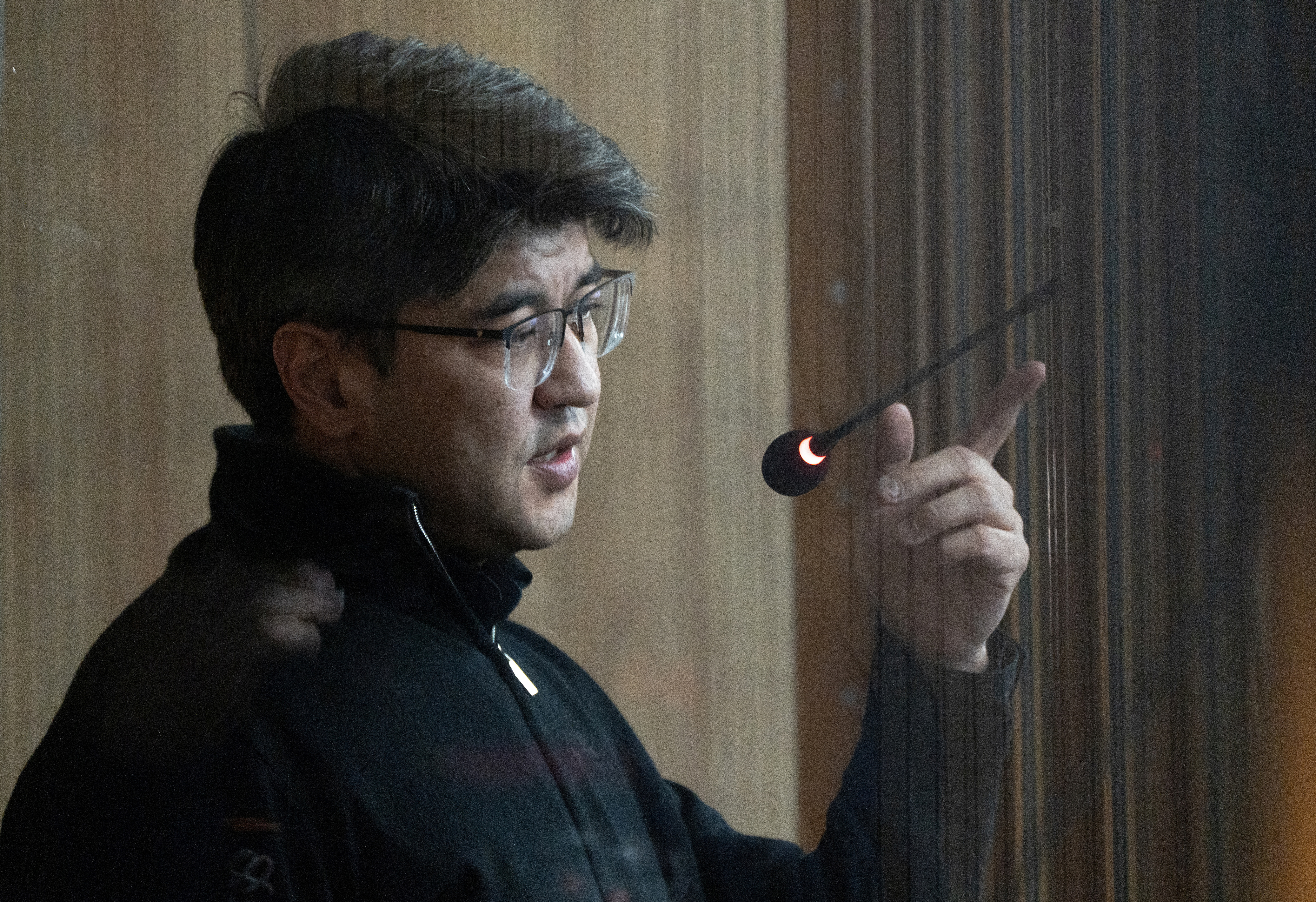
Israel-Gaza latest: IDF sacks senior officers over aid worker killings - as findings are 'damning slur' on military
The IDF has shared its findings from an investigation into strikes that killed seven aid workers, including three Britons. Meanwhile, Israel has agreed to open a crossing into northern Gaza and receive aid at an Israeli port after a "tense" call between Joe Biden and Benjamin Netanyahu.
Friday 5 April 2024 13:40, UK
- Israel-Hamas war
Please use Chrome browser for a more accessible video player
Aid worker killings
- IDF releases findings of investigation into strikes that killed seven
- Two senior IDF officers sacked for 'serious failings'
- IDF apologies are 'cold comfort', charity says
- Alistair Bunkall: Findings a damning slur on Israeli military
- Israel to open new routes for humanitarian aid into Gaza
- Mark Stone: Most significant move on aid since war began
- Biden tells Netanyahu immediate ceasefire is 'essential' and US support 'dependent' on steps to address civilian harm
- Podcast: Should the UK stop selling arms to Israel?
- Live reporting by Sharon Marris and Brad Young
There have been tense scenes in Jerusalem's Old City today on the last Friday prayers of Ramadan.
The area has sacred significance to both Muslims and Jews and has been a flashpoint for conflict before.
The Times Of Israel had reported some 3,600 police officers would be guarding the area today, with a large number of worshippers expected to attend prayers at the al Aqsa Mosque.
More than 500 police officers will be deployed across central London this afternoon to police two demonstrations related to the Middle East conflict.
Met Police said the larger of the demonstrations is organised by the Islamic Human Rights Commission, an annual event that takes place on the final Friday of Ramadan - Al Quds Day.
This march will start outside the Home Office at 3pm and head along Millbank, past parliament to Whitehall, where there will be speeches.
The second demonstration is a pro-Israel counter-protest in Parliament Square.
Commander Colin Wingrove, who is leading the policing operation, said: "The rights of people to express their views through protest must be protected and our officers will ensure they are, but anyone who abuses those rights and uses them as an opportunity to commit offences or to promote hate can expect to face police action."
Conservative MP Alicia Kearns said it was "devastating" that it had taken six months and the deaths of Western aid workers for Israel to open new routes for humanitarian aid.
The chair of the foreign affairs select committee told BBC Radio 4's Today Programme: "It's devastating that it's taken six months for us to get to a point it appears the international community is able to influence Israel's perpetration of this war.
"This has been the challenge over the last six months is that as I've gone around the world, so many people have said to me 'why can't you force Israel to do this?'. Well, Israel is our ally and we do not control them.
"And it has been the point, the phrase you have heard from interlocutor after interlocutor is that Israel is not listening. That does appear to have changed."
Ms Kearns disputed Israeli claims the air strikes were a mistake, arguing that the cars were clearly marked and their locations had been shared with the Israeli military.
She added: "It is one thing to strike one car, and then you might say: 'Well let's look at exactly what this vehicle is', but they then went on to triple tap, car by car by car."
Ms Kearns continued: "This is something that is happening on a daily basis, in terms of the attacks on humanitarians, and we are not seeing this outcry about Palestinian volunteers."
Aid staff in Gaza are determined to continue their work, despite the deaths of seven World Central Kitchen workers in an Israeli strike this week, Oxfam's food security coordinator has said.
Fidaa al-Araj said: "This is not the first incident where aid workers have been killed, even though it might be the first incident that triggered that much reaction, sadly, because they're international workers."
Ms al-Araj said that, although the seven deaths were tragic, 169 Palestinian aid workers had already been targeted and killed in the conflict, adding: "I haven't seen much reaction around the world for that."
The deaths have tested the resolve of other aid workers on the ground, she said, adding: "We know that we're targeted one way or another, we know we might leave home or the places we're taking shelter in to work and we might not get back.
"But we are determined to go on with what we're doing because I believe in what we're doing - even though it's just a drop in the ocean, sometimes it's life-saving.
"It's not easy to see that, despite our best efforts, nothing is seemingly changing... nothing is improving. This is the difficulty that we're facing."
World Central Kitchen has responded to the IDF's investigation into Israeli strikes that killed seven of the charity's workers.
Chief executive Erin Gore said Israel's apologies for the deaths "represent cold comfort".
Ms Gore said: "It's cold comfort for the victims' families and WCK’s global family."
The charity acknowledged the IDF had taken responsibility and was taking disciplinary action against those involved, saying these were "important steps forward".
"However it is also clear from their preliminary investigation that the IDF has deployed deadly force without regard to its own protocols, chain of command and rules of engagement.
"The IDF has acknowledged that our teams followed all proper communications procedures.
"The IDF’s own video fails to show any cause to fire on our personnel convoy, which carried no weapons and posed no threat."
WCK said that, without systemic change, "there will be more military failures, more apologies and more grieving families".
The charity called for an independent commission to investigate the killings, insisting the IDF was not capable of "credibly investigating its own failure in Gaza".
By Alistair Bunkall , Middle East correspondent
This wasn't an accident. It was no mistaken misfire.
The IDF cell tracking the vehicles fired lethal precision-guided missiles into each car, one after the other.
Through blurred nighttime surveillance footage, they saw what they thought was a man carrying a gun and assumed he was a Hamas fighter.
They then assumed everyone else travelling in the vehicles were also Hamas. There was no evidence for this.
They kept firing because they saw passengers still alive.
The basic failure to pass details of the aid convoy down the chain of command is a damning slur on a military that thinks of itself as being one of the best in the world.
The decision to launch air strikes with the intent of killing people, based on unsound evidence, raises deeply troubling questions of ethics in combat.
It's a sad irony that one of the only reasons World Central Kitchen were operating at night was because of their previously good working relationship with the Israeli military.
Had six of the seven killed not been foreign aid workers, whose deaths caused an international outcry, then this investigation would not have happened and the Israeli military would not have been forced to explain its actions.
How many Palestinian civilians therefore have been killed in similar, uninvestigated cases of mistaken identity, we will probably never know.
The IDF has released findings after a 72-hour investigation into three Israeli strikes on an aid convoy that killed seven workers, including three Britons.
Journalists, including our Middle East correspondent Alistair Bunkall, were briefed late last night on the key details at the Israeli defence ministry.
In summary, the IDF said, the first strike was a case of mistaken identity, and the second and third were "grave" mistakes.
The investigation has concluded that there was not enough evidence to make the convoy a legitimate target.
It was the decision to launch the second and third strikes that broke "operational procedure".
It was, in the words of the general overseeing the inquiry, "a grave mistake".
The soldiers involved have been suspended but criminal proceedings have not been opened - this will be a decision for the military advocate general.
"It's a tragedy, it's a mistake, actually it's not a mistake, it is a serious event that we are responsible for," IDF spokesman Daniel Hagari admitted.
'Misjudgement and misclassification'
On Monday night, a small team working for World Central Kitchen oversaw the unloading of the latest aid ship to arrive in Gaza from Cyprus - it was carrying 300 tonnes of food, one of the biggest shipments to date.
This was day one of what was to be a four-day operation, closely coordinated with the Israeli military and civilian authorities.
"It's a complexity because it's a four-day mission where pickups are staying in a war zone, and trucks, and they're mixed together and we have still warriors fighting," the IDF said at the briefing.
A drone operator and those overseeing the military operation mistook the volunteers for being Hamas gunmen.
"[The soldiers'] belief that the attacked vehicles were carrying Hamas gunmen was based on operational misjudgement and misclassification of the situation," the IDF said.
Details of coordination between WCK and the IDF were passed by COGAT - the Israeli government body overseeing aid into Gaza - to Southern Command, who were operating armed-drone surveillance flights overhead.
It's at this point in the chain-of-command that details of the aid convoy "stopped somewhere. We don't know where," the IDF said.
As a result, the military cell and drone pilot were not read into their task.
Timeline of events, according to the IDF
At 10pm , eight aid lorries drove south down the coast road in Gaza from the pier constructed by World Central Kitchen to a warehouse being used by the charity.
Three vehicles joined the convoy 20 minutes later.
At 10.28pm , the drone operator saw an armed person on the roof of one of the vehicles.
COGAT were notified by the IDF, but could not reach World Central Kitchen via phone.
At 10.46pm , a second gunman joined the first, at which point the IDF cell assumed them to be Hamas - but the drone operator was ordered not to strike.
Some time between 10.28pm and 10.47pm , the convoy arrived at a warehouse.
At 10.55pm , three vehicles - which we now know were carrying the seven aid workers - left the warehouse, driving south. The drone operator believed they saw an armed person getting into the car, which was a "misclassification".
Another vehicle left the warehouse, turning north - which was not part of the agreed plan, the IDF said.
The drone operator believed Hamas fighters were in the vehicles and that the aid workers had stayed at the warehouse.
As they watched the vehicles drive away from the warehouse, an IDF colonel and major signed off the order to strike.
The first missile hit at 11:09pm, hitting an armoured car. Two passengers ran out and towards the second car.
At 11:11pm , a second missile is launched, hitting the second vehicle. Some of the passengers were still alive, and ran towards the third vehicle.
At 11:13 , a third and final strike hits the last car.
The IDF has dismissed two senior military officers after the killing of seven aid workers, including three Britons, in Gaza.
The military will formally reprimand three commanders, including one who runs the Southern Command.
"The strikes on the three vehicles were carried out in serious violation of the commands and IDF standard operating procedures," the IDF said in a statement on its investigation into the strikes.
It described the attack as "a grave mistake stemming from a serious failure due to a mistaken identification, errors in decision-making".
"We express our deep sorrow for the loss and send our condolences to the families and the WCK organisation."
The officers facing dismissal or reprimand are as follows.
Dismissed: The brigade fire support commander with the rank of major and the brigade chief of staff with the rank of colonel.
Formal reprimand: The brigade commander, the 162nd Division commander, and the commander of the Southern Command.
"The IDF will learn the lessons of the incident and will incorporate them into the IDF's ongoing operations," it said.
Earlier, we reported on the UN Human Rights Council adopting a resolution calling for Israel to be held accountable for possible war crimes and crimes against humanity in Gaza.
The resolution stressed the "need to ensure accountability for all violations of international humanitarian law and international human rights law in order to end impunity".
It also expressed "grave concern at reports of serious human rights violations and grave breaches of international humanitarian law, including of possible war crimes and crimes against humanity in the Occupied Palestinian Territory".
The non-binding measure took aim at Israeli actions such as impeding access to water and limiting aid shipments. It also called on countries to stop selling or shipping weapons to Israel.
Meirav Eilon Shahar, Israel's permanent representative to the UN in Geneva, said the council had "long abandoned the Israeli people and long defended Hamas".
Twenty-eight countries approved the resolution, 13 abstained and six opposed it.
Among those opposed was the US, which said the resolution did not contain condemnation of Hamas for the 7 October attacks.
But the US did say Israel had not done enough to minimise the harm to civilians.
Michèle Taylor, US permanent representative to the council, said her country had "repeatedly urged Israel to de-conflict military operations against Hamas with humanitarian operations, in order to avoid civilian casualties and to ensure humanitarian actors can carry out their essential mission in safety".
"That has not happened and, in just six months, more humanitarians have been killed in this conflict than in any war of the modern era."
Israel's promise to open new routes for aid into Gaza is "a positive step forward after yet another tragic week", according to CARE International UK's head of advocacy and policy, Dorothy Sang.
She said allowing aid through the Ashdod Port and the Erez land crossing, as well as increased deliveries from neighbouring Jordan at the Kerem Shalom crossing, is "by far the most efficient means" of getting aid into the strip.
"Given the scale of humanitarian needs and of infrastructure destruction, we need sustained - not temporary - access, with fast-tracked screening and no delays.
“Aid workers and civilians must be protected in conflict, and humanitarian aid must not be restricted," she added.
"These are indisputable foundations of international humanitarian law - which the UK is beholden to."
Ms Sang also called for the UK government to suspend arms export licences to Israel and to support an immediate ceasefire.
Be the first to get Breaking News
Install the Sky News app for free

- Download Specs
- Download Code List
OpenTravel High Level Strategy for 2023 and beyond
API costs have become a major issue in the travel industry. The move to APIs that started 20 years ago has changed the travel market. It initially lowered connection costs and made direct connects between travel providers and channels more flexible. However, the move away from the rigor of older protocols has led to wide differences in how messages work. In addition, XML messages were created to do the same as the older messages. The processing flow didn’t change, so in most cases the legacy systems were not changed and various types of front ends (hubs, portals, gateways, etc.) translate to XML and back. This wrapping approach does not work very well now that all want to move to JSON/REST type connections, and these newer architectures work very differently. As a result, the current effort to jam JSON into existing process flows and front ends is having poor results.
This has all led to a lot of API chaos, meaning high labor costs and exceedingly long cycle times to market. The chaos refers to the large variations in how the newer APIs work and how the API producer decided to overcome the issues mentioned above. Fairly unique to the travel industry, the larger cost factor is not for the API producer, for which there are continuously improving tools, but for the API consumer. To get much of anything done in travel, you need to interoperate with others. This means everyone is hit with the problem of dealing with hundreds to thousands of APIs that do the same basic business functions but work differently. The resulting cost is a major factor in preventing travel retail from catching up to the digital capabilities of other industries.
The number of APIs encountered to do a simple trip quickly explodes. The issue is not the product, price or rules themselves, it’s how they are presented in the API.
To deal with this complicated problem in a sustainable way requires cooperation between the participants in travel retail. To support this cooperation, OpenTravel must change.
Now let’s look at how this would work. More standards are not necessarily the answer. Developers today need an example that shows them how something works and how they can copy it. This is called reference implementations. For travel, this would be end-to-end examples of common API functions and workflows. They would show best practices of API sequence, documentation, security handling, parameter passing, call backs for data like rules and so much more. All things that don’t affect or limit product team innovation to achieve market advantages. As important as API behaviors is a common understanding of the data as business objects. This may be referred to as a common data model, but it’s better to refer to it as a common syntax where not only is the data format defined, but so is the context of what the data means and what the data can do. This already exists in OpenTravel 2.0, referred to in the charts as the common model. This is all pulled together in a community sandbox.
The sandbox provides a focal point for travel industry standards bodies and trade associations. They drive the business and market direction on behalf of their members. OpenTravel shows the technical side: how to do what they want in a more consistent way across travel sectors.
You may be thinking that it will never work because these other groups will not agree on everything, but the good news is that they don’t need to. It’s OK for there to be differences between sectors or even individual providers. The critical part is that we all agree on how and where those differences are documented (documentation means in a machine-readable way like XML or JSON). Not to be used in a message but for tooling to automate handling differences. Hence the major travel industry standards bodies and trade associations can drive a common model and API practices.
What had been discussed to this point is how we get to a common data model providing syntax across travel sectors. Differences such as how an air order works versus a hotel is provided for. These differences are easily understood, and in many cases, the handling can be automated. There is still a need for differences between providers in the same sector. Part of that need is already handled in the OpenTravel common model. The common model holds all data information the community has deemed important. For each API generated, one may select from the model only what is needed for that API. If IHG wants a different combination of data than Hilton, that’s easily handled in the tooling provided.
However, what remains is the extensions to the model that the community does not deem suitable for common use. Examples include the extensions that are needed to deal with a specific legacy backends (PMS. CRS, GDS, etc.). Some providers may make extensions they feel are a competitive value. Today these extensions are invisible behind the firewall of providers. Retail partners only see them when they provide documentation and are eventually allowed access through the firewall. This effort itself, to provide documentation and allow access, is expensive.
The alternative is to provide a service in the OpenTravel common model for private extensions. Consider a scenario where I am a commuter airline or app developer startup that wants to direct book with a hotel provider. I can see what is in the common model for hotels, but it does not provide all the necessary information needed to work with this hotel company. They provide me access to their extensions hosted in the same site and sandbox where the common model is. Now I know all I need is to connect. Further, I can mock test all APIs without bothering the dev and security teams at the hotel. Once testing is done and commercial agreements are in place, then the effort moves into acceptance testing requiring firewall access. The hotel company has deferred nearly all costs until they can witness via a POC that the potential partner can deliver. Note this also acts as a means for the hotel company to distribute API updates. API consumers can pick up the changes and test when they are ready. Also note that access to a real test system at the hotel can be set up thru the sandbox. This further reduces impact on hotel dev and security teams as the needed infrastructure is in place and reusable.
What follows is a similar diagram to one before showing the standards bodies and trade associations. What’s different is the direct participation of the API providers as they can extend the model as needed to support real world scenarios that are implementable.
We’re in the home stretch of the strategy. This publication mostly talks about the first phase of modernizing travel retail via cooperation. Further steps are enabled by phase one. A big step is to move into offer and order management that is totally cloud based. We’re not talking about an individual provider or channel using the cloud for caching. This will be more expansive and cooperative to provide sub second response time around the globe. Next, or in parallel, would be the exploitation of AI/ML. Use of AI/ML is still fairly limited to specific use cases like sorting out which fares are worth calculating for air availability calls. Some are working on use of AI/ML to support personalization. However, the latter is limited to private channels and not at a trip level. Not at scale anyway. The issue is lack of reliable data and cross provider/channel identity management. The first phase of cooperation thru OpenTravel provides a foundation these and many more issues.
API chaos is costing the travel industry large amounts of money, adds time to deployment, and is getting worse with the moves to JSON/REST. The chaos locks a lot of travel content out of the market as it is too expensive to connect. There is a large impact on the extension of AI/ML solutions for travel retail, because of poor data quality. This is directly due to the lack of a common understanding of data syntax. This can only be resolved as a community. Keep in mind not everyone needs to agree on the meaning of every data element. What is needed is to agree to disagree, and to document in a common way what the differences are.
Investing is OpenTravel is not a payment for messages and a model anyone can download anyway. Investing in OpenTravel is about opportunity for cost avoidance and revenue enhancement far greater than the investment. OpenTravel was founded because it was far cheaper to work together to define XML messages than do it individually. The same is true for APIs today. It will be far cheaper to deploy and consume APIs if the industry players cooperate on the noncompetitive aspects of how APIs work. Lowering API costs would unlock immense amounts of travel content and allow AI/ML technology to live up to the hype. Please support the OpenTravel mission. It makes business sense to do so.
If you want to get the pdf file, click here .
- Specifications
- Current Events
- Announcements
- Download OTM Tool
Follow Us On:
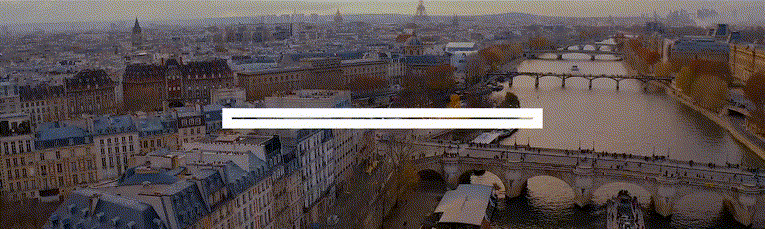
COMMENTS
Open Travel Data (OPTD) provides a collection of transport, travel and leisure related data. The project makes extensive use of already existing data sources such as Geonames and Wikipedia , and adds some glue around those (e.g. links). All data sets are as carefully maintained as possible, and kept up-to-date by the OPTD team, which we would ...
Open Travel Data (OPTD) provides a collection of transport, travel and leisure related data. The project makes extensive use of already existing data sources such as Geonames and Wikipedia, and adds some glue around those (e.g. links).. All data sets are as carefully maintained as possible, and kept up-to-date by the OPTD team, which we would be glad to welcome you on!
About OpenTravel. The OpenTravel Alliance provides a community where companies in the electronic distribution supply chain work together to create an accepted structure for electronic messages, enabling suppliers and distributors to speak the same interoperability language, trading partner to trading partner. Learn More.
Stravl-Data is the world's largest, open-sourced data set of travel preferences. Through a user-facing website, Stravl gathered 80,301 travelers' vacation preferences. Users were asked to fill out a brief form on their ideal vacation preferences (such as expected experiences, scenery, and activity level) and logistical constraints (such as ...
opentraveldata Public. Collection of open data related to (at least) travel, transport, tourism. Awk 225 96 14 (2 issues need help) 0 Updated 11 hours ago. python-opentraveldata Public. Python wrapper around OpenTravelData (OPTD) Python 2 0 1 2 Updated on Dec 5, 2023. quality-assurance Public. Quality Assurance (QA) for Open Travel Data (OPTD ...
About data.world; Terms & Privacy © 2024; data.world, inc ... Skip to main content
And there is Open Data for all kind of travel purposes, regardless of whether you are going to visit a city, explore nature or relax on the beaches. For instance, 695 datasets are found for "camping" on the European Data Portal. An example is a dataset originating from Saarland, one of the sixteen Bundesländer of Germany.
This is the master repository of the Open Travel Data (OPTD) project.\nFor backwards compatibility reasons, all changes are synchronized back to the\nold repository. \n Licensing \n. The data curated and/or generated by the OPTD project are governed by the\nCC-BY license. \n With OPTD own generated/curated data, you are free to \n \n
Open Data plays a key part in promoting the tourism sector. The company outdooractive manages many tourist data with the open central database solution. These include events, current news, region and place descriptions, tours and activity trail networks, accommodation reviews, excursion destinations and sights, and stories, pictures or videos.
Measurement(s) travel-related behavior • travel-related attitude Technology Type(s) Survey Factor Type(s) temporal interval Sample Characteristic - Organism Homo sapiens Sample Characteristic ...
Check that the data files have been properly downloaded, and that their sizes are as expected (40 to 50 MB for the IATA/ICAO data file and 4 to 5 MB for the UN/LOCODE data file): >>> myOPTD . validateFileSizes () True
That repository features scripts to check the quality of the data files produced by the Open Travel Data (OPTD) project. Though it is not well maintained, that project should produce a Quality Assurance (QA) dashboard, much like Geonames' one. See the Geonames use case on the Data Quality reference page for more details.
Built by the Travel Industry, For the Travel Industry. Since 2001, OpenTravel has built, promoted and evolved the data messaging standard for the travel industry. The combined efforts of all of our stakeholders over the years have resulted in a unifying specification that has been implemented by hundreds of travel companies - representing ...
1. Documentation. OpenTravel provides the Specifications for voluntary use by individuals, partnerships, companies, corporations, organizations, and other entities at their own risk.; The Specifications and any OpenTravel supplied supporting information, data, or software in whatever medium in connection with the Specifications are referred to ...
That project makes an extensive use of already existing data sources such as Geonames and dbPedia, and adds some glue around those (eg, links). Tags. boost boost_python c++ database framework free geo geography geolocation geospatial geotools i18n i18n_(internationalization) javascript key-value mysql nosql python search text travel
Open data discoveries for MTA enthusiasts. MTA. Updated March 25, 2024 5:00 p.m. It's been a whirlwind month for MTA Open Data! Our team has been busy celebrating NYC Open Data Week, which happens annually in March. We've published additional resources, extra blog posts, and released our annual update to the Open Data Plan.
The OTDS association. The "Verein zur Förderung eines offenen touristischen Datenstandards e.V." (Association to promote an Open Travel Data Standard, OTDS e.V. in Berlin, Germany) specifies and develops the data format OTDS for the travel industry. OTDS is based on open, nondiscriminatory, and standardized technologies.
Hence there is no doubt that unfavorable exchange rates and exchange rate volatility can lead to changes in travel patterns, especially in vacation scheduling. Such an argument has been confirmed by Webber and the World Travel and Tourism Council . As per the existing theory, the exchange rate affects tourism in two different ways.
In conclusion, open data offers a wealth of opportunities for enhancing traffic control management. By leveraging insights from open data and utilising available datasets, authorities can implement effective strategies to reduce congestion, improve travel times, and ultimately create more efficient and sustainable transportation systems.
Download Open Travel Data for free. Framework (scripts, configuration, code) to build free and public services around travel and leisure data. That project makes an extensive use of already existing data sources such as Geonames and dbPedia, and adds some glue around those (eg, links).
OpenTravel's 2.0 is an object-oriented model, a model that more closely captures data in a way that developers work, and that is better adaptable to future development. Objects combine data and behavior, and use structure to define relationships. Once objects are established, they can be used again and again, but it's also a simple process ...
The Open Travel data Access Protocol (OTAP) project makes the traffic data from European Traffic Information Centers available to Service Providers through the Internet using standard technology and common formats. It also looks to facilitate the organizational problems encountered in accessing this data, by making procurement information ...
If the issue persists, it's likely a problem on our side. Unexpected token < in JSON at position 4. keyboard_arrow_up. content_copy. SyntaxError: Unexpected token < in JSON at position 4. Refresh. This dataset provides detailed information on various trips.
Hudi's origins as a solution to Uber's data ingestion challenges make it a good choice when you need to optimize data processing pipelines. In contrast, Netflix developed Iceberg to simplify the big data management issues of the Hadoop and Hive ecosystem. As such, migrating to Iceberg tables is ideal for storing large datasets in a data lake.
Usage. Launch a Python interpreter: $ python. >>>. Import the opentraveldata library: >>> import opentraveldata. Specify a variable, say myOPTD, as a handle on the OPTD library. With the default local directory for the data files, that is /tmp/opentraveldata. >>> myOPTD = opentraveldata.
The 7.4 magnitude earthquake that killed at least nine people and injured hundreds Wednesday, is the strongest to hit Taiwan in 25 years. Over the last 50 years, the island has experienced a total ...
In response the OpenTravel Alliance (OTA) and the OpenAPI Initiative (OAI) will work together to focus on API conventions and standards, not just messages. Within OAI, there is now a special interest group to focus on travel issues (#sig-travel). The travel SIG will be the conduit for the needs of the travel industry that pertain to the OpenAPI ...
LONDON, March 26 (Reuters) - A major bridge collapsed in the U.S. port of Baltimore in the early hours of Tuesday after being struck by a container ship, plunging cars into the river below.
Israel has agreed to open a crossing into northern Gaza and receive aid at an Israeli port after a "tense" and "strained" phone call between Joe Biden and Benjamin Netanyahu. The IDF is set to ...
The chaos locks a lot of travel content out of the market as it is too expensive to connect. There is a large impact on the extension of AI/ML solutions for travel retail, because of poor data quality. This is directly due to the lack of a common understanding of data syntax. This can only be resolved as a community.